Open Access
ARTICLE
Night Vision Object Tracking System Using Correlation Aware LSTM-Based Modified Yolo Algorithm
1 Department of Computer Science and Engineering, K.L.N. College of Engineering, 630612, Tamilnadu, India
2 Department of Electronics and Communication Engineering, Thiagarajar College of Engineering, Madurai, 625015, Tamilnadu, India
* Corresponding Author: R. Anandha Murugan. Email:
Intelligent Automation & Soft Computing 2023, 36(1), 353-368. https://doi.org/10.32604/iasc.2023.032355
Received 15 May 2022; Accepted 28 June 2022; Issue published 29 September 2022
Abstract
Improved picture quality is critical to the effectiveness of object recognition and tracking. The consistency of those photos is impacted by night-video systems because the contrast between high-profile items and different atmospheric conditions, such as mist, fog, dust etc. The pictures then shift in intensity, colour, polarity and consistency. A general challenge for computer vision analyses lies in the horrid appearance of night images in arbitrary illumination and ambient environments. In recent years, target recognition techniques focused on deep learning and machine learning have become standard algorithms for object detection with the exponential growth of computer performance capabilities. However, the identification of objects in the night world also poses further problems because of the distorted backdrop and dim light. The Correlation aware LSTM based YOLO (You Look Only Once) classifier method for exact object recognition and determining its properties under night vision was a major inspiration for this work. In order to create virtual target sets similar to daily environments, we employ night images as inputs; and to obtain high enhanced image using histogram based enhancement and iterative wiener filter for removing the noise in the image. The process of the feature extraction and feature selection was done for electing the potential features using the Adaptive internal linear embedding (AILE) and uplift linear discriminant analysis (ULDA). The region of interest mask can be segmented using the Recurrent-Phase Level set Segmentation. Finally, we use deep convolution feature fusion and region of interest pooling to integrate the presently extremely sophisticated quicker Long short term memory based (LSTM) with YOLO method for object tracking system. A range of experimental findings demonstrate that our technique achieves high average accuracy with a precision of 99.7% for object detection of SSAN datasets that is considerably more than that of the other standard object detection mechanism. Our approach may therefore satisfy the true demands of night scene target detection applications. We very much believe that our method will help future research.Keywords
Cite This Article
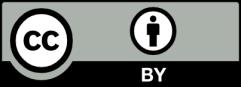
This work is licensed under a Creative Commons Attribution 4.0 International License , which permits unrestricted use, distribution, and reproduction in any medium, provided the original work is properly cited.