Open Access
ARTICLE
Explainable Heart Disease Prediction Using Ensemble-Quantum Machine Learning Approach
1 College of Computer and Information Sciences, Princess Nourah bint Abdulrahman University, P.O.Box 84428, Riyadh, 11671, Saudi Arabia
2 Department of Information Technology, College of Computer and Information Sciences, Princess Nourah bint Abdulrahman University, P.O.Box 84428, Riyadh, 11671, Saudi Arabia
3 Department of Computer Science, College of Computer and Information Sciences, Princess Nourah bint Abdulrahman University, P.O.Box 84428, Riyadh, 11671, Saudi Arabia
* Corresponding Author: Souham Meshoul. Email:
Intelligent Automation & Soft Computing 2023, 36(1), 761-779. https://doi.org/10.32604/iasc.2023.032262
Received 12 May 2022; Accepted 24 June 2022; Issue published 29 September 2022
Abstract
Nowadays, quantum machine learning is attracting great interest in a wide range of fields due to its potential superior performance and capabilities. The massive increase in computational capacity and speed of quantum computers can lead to a quantum leap in the healthcare field. Heart disease seriously threatens human health since it is the leading cause of death worldwide. Quantum machine learning methods can propose effective solutions to predict heart disease and aid in early diagnosis. In this study, an ensemble machine learning model based on quantum machine learning classifiers is proposed to predict the risk of heart disease. The proposed model is a bagging ensemble learning model where a quantum support vector classifier was used as a base classifier. Furthermore, in order to make the model’s outcomes more explainable, the importance of every single feature in the prediction is computed and visualized using SHapley Additive exPlanations (SHAP) framework. In the experimental study, other stand-alone quantum classifiers, namely, Quantum Support Vector Classifier (QSVC), Quantum Neural Network (QNN), and Variational Quantum Classifier (VQC) are applied and compared with classical machine learning classifiers such as Support Vector Machine (SVM), and Artificial Neural Network (ANN). The experimental results on the Cleveland dataset reveal the superiority of QSVC compared to the others, which explains its use in the proposed bagging model. The Bagging-QSVC model outperforms all aforementioned classifiers with an accuracy of 90.16% while showing great competitiveness compared to some state-of-the-art models using the same dataset. The results of the study indicate that quantum machine learning classifiers perform better than classical machine learning classifiers in predicting heart disease. In addition, the study reveals that the bagging ensemble learning technique is effective in improving the prediction accuracy of quantum classifiers.Keywords
Cite This Article
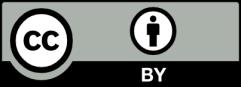
This work is licensed under a Creative Commons Attribution 4.0 International License , which permits unrestricted use, distribution, and reproduction in any medium, provided the original work is properly cited.