Open Access
ARTICLE
Effective Diagnosis of Lung Cancer via Various Data-Mining Techniques
1 Bharathiar University, Coimbatore, 641046, India
2 Bharathi Women’s College, Chennai, 600108, India
3 Faculty of Electrical and Computer Engineering, University of Engineering and Technology, Peshawar, 25000, Peshawar, Pakistan
4 Department of Information Technology, College of Computer and Information Technology, Taif University, P.O. Box 11099, Taif, 21944, Saudi Arabia
5 Department of Information Technology, Al Baha University, P.O. Box 1988, Al Baha, 65431, Saudi Arabia
* Corresponding Author: Irshad Hussain. Email:
Intelligent Automation & Soft Computing 2023, 36(1), 415-428. https://doi.org/10.32604/iasc.2023.032053
Received 05 May 2022; Accepted 29 June 2022; Issue published 29 September 2022
Abstract
One of the leading cancers for both genders worldwide is lung cancer. The occurrence of lung cancer has fully augmented since the early 19th century. In this manuscript, we have discussed various data mining techniques that have been employed for cancer diagnosis. Exposure to air pollution has been related to various adverse health effects. This work is subject to analysis of various air pollutants and associated health hazards and intends to evaluate the impact of air pollution caused by lung cancer. We have introduced data mining in lung cancer to air pollution, and our approach includes preprocessing, data mining, testing and evaluation, and knowledge discovery. Initially, we will eradicate the noise and irrelevant data, and following that, we will join the multiple informed sources into a common source. From that source, we will designate the information relevant to our investigation to be regained from that assortment. Following that, we will convert the designated data into a suitable mining process. The patterns are abstracted by utilizing a relational suggestion rule mining process. These patterns have revealed information, and this information is categorized with the help of an Auto Associative Neural Network classification method (AANN). The proposed method is compared with the existing method in various factors. In conclusion, the projected Auto associative neural network and relational suggestion rule mining methods accomplish a high accuracy status.Keywords
In the western world, the main reason for death is lung cancer. It is established using the astonishing statistical data available yearly from the American Lung Cancer Society. If the lung cancer was diagnosed in the early stage, the chance of improving the survival rate can go up from 14% to 49% as per their calculation. Around the globe, one of the major reasons for death is lung cancer as per reports. It is also the familiar reason for mortality in men and the 2nd conjoint in women [1,2]. Lung cancer is majorly categorized as “Non-Small Cell Lung Cancer (NSCLC) and the Small Cell Lung Cancer (SCLC) [3] along with NSCLC being the main form. Squamous Cell Carcinoma (SCC) and Adenocarcinoma are the two chief forms of NSCLC underwriting for 80% of NSCLC cases [4–6]. The chief approaches to the analysis of lung cancer are the therapeutic picturing investigation, lump indicators recognition, negligibly invading mediation investigation, and sputum cytology investigation presently [7]. Lately, lung cancer information from SEER was utilized to concept prognostic models for lung cancer survival after six months, nine months, one year, two years, and five years of identification with the help of numerous machine methods [8]. Additionally, new group research established a possible negative impact on lung cancer (LC). Though, indication linking acquaintance to external pollution in air with the jeopardy for LC is yet restricted, specific in some countries in Asia. In the preceding study, we recommended the danger only in people who don’t smoke but not in people who are present smokers or previous smokers [9]. There are many dissimilar observation methods that could be non-invading or biopsy methods, presently utilized for primary LC recognition. A few among them are emphasized. The communal observation methods for LC are traditional picturing methods such as “chest radiography (film or digital) and computed tomography (CT)”. Digital radiography gives improved dissimilar resolution with the same or superior spatial resolution if associated with traditional radiography methods [1].
The article evaluates mine respiration schemes and pollution in air influences on Merelani’s 4000 artisanal tanzanite miners was projected by Laurent Paul Mayala et al. [10]. The high amount of “Carbon Monoxide (CO)” quantified by the Ontario Ministry of Labour (2015) was advanced by 2.5 times at the 5 nominated mines where it averaged 66.2 ppm. As per the mine regulation of America, the average amount of reparable dust should be 2 mg dust/m3 but at present, the amount is 8 mg dust/m3. During the years 2005 to 2014, 29% of the death was due to asphyxiation in Merelani. These accidents occurred because of the entry into temporary stops which is unauthorized. 6.6% of miners have TB and many miners have silicosis and other diseases related to the lungs. The analysis highlights the prerequisite of training, usage of dust masks, enduring to safe mining practices, dust control approaches, gas monitoring, forced fans, pre and post medical checks, and as the events progress well-being and miner’s welfare at the arena.
Exact and certain colon subdivision from CT pictures is a vital stage of numerous medical implementations in CT colonography, comprising CAD of colon polyps, 3-D virtual flythrough of the colon, and then the prone/supine listing. Additional-colonic constituents can be considered into 2 forms on the basis of their 3-D relation to the colon: disconnected and connected additional colonic constituents (DEC & AEC, correspondingly). In this article, Xiaoyun Yang et al. [11], have projected graph deduction approaches to eliminate additional colonic constituents to attain huge standard separation. We primarily crumble individual 3-D air-packed things into a group of 3-D places. A classifier qualified with place-degree components can be utilized to recognize the colon places from non-colon places. Following eliminating palpable DEC, we eliminate the residual DEC by illustrating the worldwide anatomic edifice with an a priori topological restraint & resolving a graph deduction issue by means of semantic data given by a multiclass classifier.
Public health influences of surface coal mining were proposed by Ref. [12–14]. Specific attention is remunerated to the current indication for a form of surface mining experienced in the United States, specifically mountaintop elimination mining. Studies from other portions of the world are also temporarily defined. Evidence is offered that documents epidemiological disease decorations for populations existing in immediacy to surface mining. Ecological indication has displayed that surface waters then biota is damaged by mountaintop elimination; while other ecological studies have revealed water and air pollution occur in residential areas close to mining. Studies that are capable of unswervingly linking ecological exposure, dose, and biological influence are immediately needed. Though direct mechanistic links are not much assumed, the weight of the indication reinforces preceding science-based calls to cease mountaintop removal mining because of its ecological and public health jeopardies.
The associations between regional pollution in air and city design with importance on city segmentation were given by [13–16]. Utilizing a sole data of 249 “Large Urban Zones (LUZ)” through Europe, a Bayesian Model “Averaging model selection” technique is engaged to classify the causes of in-LUZ amount of 3 air pollutants: NO2, PM10, and SO2. These are complemented by numerous guides of land cover and a group of information on numerous monetary, statistical data & atmospheric elements that might elucidate the difference in pollution in the air. The solutions of this investigation assist the supposition that city design has an important impact on pollution amount.
Lung cancer is the furthermost communal cancer globally & the 5th main corporate reason for mortality worldwide. Its occurrence endures upsurging, particularly within “Low & Middle Income Countries (LMICs)” that have restricted the ability to discourse the increasing prerequisite for intervention. Veerachamy, et al. [15], have anticipated, that protection for LC management usually includes radiation therapy (RT) that acts as a significant beneficial part in the therapeutic-intent intervention of beginning level to nearby progressive disease, and also in assistance. The substructure, instrument, and HR obligatory for RT may be restricted in LMICs. Though, this descriptive analysis deliberates the extent of the issue of LC in LMICs, the part of RT automation in LC intervention, and the RT ability in emerging countries. Numerous international initiatives are presently in process and characterize significant initial stages toward increasing RT in LMICs to treat LC.
A fine-grained Vehicle type classification (VTC) method using a lightweight convolutional neural network with feature optimization and joint learning strategy was given by [17,18]. A lightweight convolutional network with feature optimization is designed and used depthwise separable convolution to reduce network parameters. To obtain the important degree of each feature channel automatically through the sample-based self-learning, which can improve recognition accuracy with fewer network parameters growth.
Sensor data validation and fault diagnosis using Auto-Associative Neural Network for HVAC systems is given by [19]. Sensor data validation and fault diagnosis for HVAC systems is developed by a data-driven approach using the system’s normal operation data and without the need for the knowledge of the mathematical model of the system. It is based on an Auto-Associative Neural Network (AANN) that is structured and trained to construct an input-output mapping model based on data dimensionality reduction that is capable of validating sensor measurements in terms of sensor error correction, missing data replacement, noise filtering, and inaccuracy correction.
Multiple Fault Diagnosis of Aeroengine Control System Based on Auto associative Neural Network is given by [20–23]. In order to obtain a fault diagnosis system with strong robustness and high detection rate, we design the Auto Associative Neural Network (AANN) group to complete the detection and isolation of Aeroengine sensor faults and component faults, as well as the reconstruction of sensor faults.
• Our mission was to assess the air everyone breathes rather than focus on exact air pollutants. The consequences from the revised studies point in a similar direction: the risk of emerging lung cancer is suggestively augmented in people unprotected from air pollution.
• Connotation rule mining is an important approach. Though, as large amounts of association principles are frequently from time to time simple.
• Numerous organizations rule mining is typically much more expensive. To overcome these disadvantages, we projected the relational associative rule mining method.
In our projected methodology (as mentioned in Fig. 1), we will familiarize ourselves a data mining on lung cancer by air pollution. Our methods comprise pre-processing, data mining, testing and assessment, and knowledge discovery. Our principal phase is data cleaning, is this phase is used to eliminate the noise and immaterial data from the collection. The subsequent phase is a data assimilation phase at this phase, multiple information sources, repeatedly heterogeneous, may be collected in a common source. When the data integration procedure is accomplished then we will go for a selection procedure in this data selection phase the information applicable to the investigation is categorical and recovered from the information collection. Subsequent phases are a data consolidation phase in this phase the designated dates are distorted into forms suitable for the mining process. After this phase, we will familiarize ourselves a data mining to use a relational association rule mining method are implemented to abstract designs potentially beneficial. Then the subsequent stage is a pattern assessment phase in this phase the stimulating patterns of representative knowledge are recognized on the basis of specified measures. Then the final phase is a knowledge illustration is a final phase in that the exposed knowledge is visually characterized by the consumer. In this step, amended AANN classification methods are used to aid users to comprehend and understand the data mining solutions.
Figure 1: Proposed data mining model for lung cancer caused by air pollution
Data pre-processing in Data Mining is one of the most significant facts within the well-known knowledge invention from the data processor. Data that were immediately taken from the origin will have errors, inconsistencies, or most significant, it is not prepared to be considered for a data mining method. The disturbing numeral data in the industry, recent science, calls, and business applications to the requirement of additional complex tasks are analysed. In Data pre-processing, it is feasible to modify the adverse into feasible. Data pre-processing contains detecting, and data reduction techniques, decreasing the complexity of the information, or noisy elements from the information.
Data integration is the procedure of technical and business procedures utilized to syndicate information from dissimilar sources into meaningful and valuable data. A comprehensive data integration result offers trusted information from the variability of sources. Possibly the most well-known application of data integration is constructing an enterprise’s information warehouse. The advantage of a data warehouse empowers a business to accomplish analyses on the basis of the information in the data warehouse. Data integration syndicates information from various origins to create a coherent information stock. Metadata, correlation investigation, information dispute observation, and resolution of semantic heterogeneity subsidize smooth information combination. It is likely that your investigation will include data integration that pools information from various origins into a coherent information store as in data warehousing. These resources may include multiple database information cubes or flat files. This data integration is the main procedure of our study it is mostly utilized for syndicating the data from multiple sources into the corporate source.
Data selection is well-defined as the procedure of responsible for the appropriate information type and source, and also appropriate instruments to gather information. Data assortment leads to the actual practice of information assortment. This description discriminates information selection from selective information reporting and interactive/active information selection. The procedure of selecting appropriate information for a research project can influence integrity. The main objective of information selection is the purpose of appropriate information type, resource, and instrument(s) that permit agents to sufficiently answer research questions. Data selection stage the data relevant to the investigation is absolute and recovered from the information collection. In an information selection procedure, it will select the relevant data and eradicate the inappropriate data.
Data association denotes the collection and integration of information from multiple sources into a solitary destination. During the period of this procedure, dissimilar information sources are put together or combined, into a single information store. As information comes from a broad range of sources, consolidation permits organizations to more effortlessly present information, while also simplifying effective information investigation. Data consolidation methods decrease inadequacies, such as information duplication, costs associated with reliance on multiple databases, and multiple information management points. We can describe the term information consolidation as a procedure in which a massive amount of data is congregated, stored, and joined into a single manipulative file, stereotypically inside a database. Typically, consolidation is proficient with the help of a computer with distinct software implementations, namely a spreadsheet or a database program, premeditated to pleat, establish and store information.
From enormous information repository data mining or knowledge, discovery denotes the process of finding associated interesting pattern data. It as well denotes the stage in the knowledge discovery procedure that utilizes special algorithms to be capable to identify interesting patterns in the information. These interesting designs are subsequently examined to give up knowledge. Manner, Data Mining signifies the task of getting or mining information from mega datasets. Data mining is a device to analyze information, and it can aid consumers to recognize the substantial and the imperative of the relationships found in the information. Data mining is the procedure of automatically amassing large volumes of information with the objective of finding unseen patterns and investigating the relationships between frequent types of information to progress predictive models. The classification methods and prediction are two forms of information analysis that can be utilized to abstract models describing significant information classes or to predict future data trends.
Relational Association Rule Mining
The principle will be fashioned on the basis of the preferred features or metrics. Relational suggestion rule mining is engaged to yield the principle of the recommended technique. Relational suggestion rule mining is a method that is the addition of ordinary suggestion rule mining. Assume that records in the interpersonal model Rrm = {r1, r2….rN}, where each record comprises of M attributes (x1, x2….xM). The length of a relational reminder rule can be at most equal to the number of the qualities explaining the information. The relational suggestion rule mining can be designated relations namely, less or equal (≤), equal ( = ), greater or equal (≥). In some instances, the quantified procedure of relational association rule mining is made unfurnished. Replicate on one sample dataset, it’s discovered in Tab. 1, we are supposing A, B, C, and D are the software metrics. The rule will be formed on the basis of these metrics.
The principle will be fashioned on the basis of the relation of each feature after the feature assortment. Currently the length of the principle is same to the number of elements in the sample dataset. A scarce of the principles are quantified beneath,
Rule 1: A > B
Rule 2: A < C
Rule 3: A = D
Rule 4: A > B < C
Rule 5: A = D > B
Rule 6: A = D > B < C
Lastly these principles are enhanced to get the best rule. The projected technique usage adapted artificial bee colony algorithm for rule optimization. The stage-by-stage process of adapted artificial bee colony algorithm is mentioned below.
Testing and Evaluation is the procedure by that a system or apparatuses and compared against necessities and stipulations via testing. The solution is assessed to evaluate progress of design, supportability, and performance. Validation is “the process of assessing how well the mining models perform against real data”. It is essential that to authenticate the proposed mining copy by knowing their standard and traits before to position into a manufacturing surrounding.
Knowledge Discovery in Databases (KDD) the notion is that by routinely selecting via great quantities of information it should be imaginable to abstract nuggets of knowledge. The KDD and Data Mining are frequently utilized interchangeably. KDD is the procedure of turning the low-level information into high level knowledge. Henceforth, KDD refers to the nontrivial extraction of implied, previously unknown and possibly useful data from information in databases. While datamining and KDD are frequently preserved as equivalent words but in real data mining is a significant stage in the KDD procedure.
Auto-Associative Neural Network
The Neural Networks (NN) signifies the data working patterns that duplicate the way in which the biological nervous schemes work on the information. Overall, there are 4 basic sections of NN like the processing units, activation performances, weighted interconnections, and the activation principles. The “Auto-associative neural networks (AANN)” establish the network pattern in that the network is skilled to recollect the inputs as the results hence safeguarding that the networks are functioning to estimate the data as outputs when novel data are presented. The consistent networks have been expansively engaged in a multiplicity of implementations. An auto-collaborative network cipher called as an “auto-encoder” incorporates data and result layer with the number of data being correspondent to the number of outputs; consequently, it has accomplished the name ‘auto-associative’. Along with these 2 layers, there is a thin unseen layer. It is exceedingly vital that the unseen layer efficiently engages a lower dimension, to apply the encoding and decoding measures. The hidden layer inclines to reconstruct the data to equal the results by meaningfully decreasing the flaws amongst the data and result with the blend of novel data. The thin secret layer uses important effort on the network to reduce any redundancy that is probable to crop up in the information during authorizing the network to recognize essential information. Fig. 2 efficiently pictures the edifice of an AANN. As far as the present investigation is disturbed, only one unseen layer is engaged as it is recognized lack of any uncertainty that a lone unseen layer network is experienced to estimate any intact multivariate performance to any satisfactory amount of accuracy. The number of junctions in the unseen layer is unambiguous by the ability of the network to imprecise the flaw performance.
Figure 2: Structure of FFBNN classifier for the proposed work
Neural Network Working Steps
1) Set loads for all the neurons excluding the neurons in the input layer.
2) Progress the neural network with the abstracted attributes {A1, A2, A3, A4, A5} as the input units, HUa Hidden units, and age f as the output unit.
3) The computation of the recommended Bias performance for the input layer is,
The activation performance for the output layer is assessed as
4) Identify the learning error as presented beneath.
where,
- learning rate of FFBNN.
- Desired outputs.
- Actual outputs
The projected Data mining in lung cancer by air pollution is applied in the platform Java. The experimental solution and the function of the projected system are mentioned below in detail.
There are numerous customs of significant ecological study, but we investigate how these atmospheres were distressing the human health. In this atmosphere, we have inhaled something like air, water, and food from our environments. Every day, we have faced chemicals, radiation, microbes also a physical world. All these things distress our health.
At this time, we occupied a particle matter is PM2.5 fine particulates, fine particulate matter or fine particles (PM2.5), are minute elements or droplets in the air that have sole physical properties including shape, volatility, and density. Generally, this size fraction can be believed of as having a dimension of roughly two- and one-half microns across. PM2.5 theoretically denotes particles that have an aerodynamic diameter of 2.5 micro meters and less. There are external & internal resources of minute elements. The Outer, minute particulates principally come from on-road and off-road vehicle emissions; other major reasons are the burning of fuels and natural fire. Minute particulates are created from the combination of gases in the air from the sources like power plants. These chemical combinations can happen at a far distance from the innovative emission sources. These particles were mostly exaggerated in the lungs also the 10 micro meters can annoy the eyes, nose, and throat all these are extended into the lung, and being uncovered to fine particulates can source lung cancer.
4.2 Performance Analysis of the Proposed Methodology
An investigational solution is utilized to assess the efficiency of the projected schemes and to explain theoretical & practical expansions of these schemes.
Tab. 2 exposures the accuracy attained for all valuations. At this time, we have attained accuracy based on the support values. The equivalent value for closing the support value 0.7 is proficient and attains an accuracy is 80%. Then the support value is 0.6 proficient to obtain 75% accuracy. Then the equivalent value for closing the 0.5 support value is recommended to attain an accuracy is 70%. Subsequently, the equivalent value for finishing the support value 0.4 is proficient and obtains accuracy is 60%. The graphical figure is established in Fig. 3. Tab. 2 displays the accuracy obtained for our projected study.
Figure 3: Graph for accuracy obtained for our proposed method
Tab. 3 elucidates the no of principles are produced for a study based upon a support value. In our projected methodology we have used a relational suggestion rule mining to produce rules these exclusive rules are taken from the procedure are 5, 5, 7, and 17 based on our support values they are 0.7, 0.6, 0.5, and 0.4. Our investigation has generated a principle the range that has revealed in Tab. 3 and the graphical diagram is established in Fig. 4.
Figure 4: Graph for unique rules obtained for our proposed study
Tab. 4 elucidates the total no of Time taken for our study constructed upon a support value. We will declare the memory space engaged values in Tab. 4 a memory space engaged for each and every procedure is provided here primarily the support value for our procedure is 0.7, 0.6, 0.5, and 0.4, on the basis of these principles they have taken a time namely 51000, 50457, 62544 and 85215. Our investigation has engaged a low level of time that has been displayed in Tab. 6 and the graphical diagram is established in Fig. 5.
Figure 5: Graph for time taken for our proposed study
In Tab. 5 elucidates the total of memory space occupied for a study based upon a support value. We will offer a memory space engaged for each and every procedure given here firstly the support value for our procedure is 0.7, 0.6, 0.5, and 0.4, on the basis of these principles we have the memory they are attained the 2134185, 2385414, 2578416 and 2954841. Our research has employed a low level of our memory space that has revealed in the table and the graphical diagram is established in Fig. 6.
Figure 6: Graph for memory space taken for our whole process
4.3 Comparison of Proposed Methodology with the Existing Methods
If we associate a projected Auto Associative Neural Network to a prevailing neural network our projected methodology will give a better accuracy that is high and the available technique will give low accuracy solutions which are illustrated in Tab. 6. We have engaged accuracy values based upon a support value. The provided support values are 0.7, 0.6, 0.5, and 0.4.
In assessment with the neural network, the algorithm provides very fewer accuracy values. In our projected dataset we achieve Henceforth the efficacy approaches computation exposed that our Proposed technique is more successful than the Existing technique it has graphically characterized in Fig. 7. For the dataset, we achieved 80%, 75%, 70%, 60%, of accuracy based upon the support value 0.7, 0.6, 0.5 and 0.4. In a similar dataset, we have applied the neural network we have attained the low accuracy accomplished values are 75%, 64%, 66%, and 53%. Thus, we can demonstrate that our anticipated Auto associative neural network algorithm-based classification can outpace the state-of-art works by effectually categorizing the discovered knowledge.
Figure 7: Graph for comparison of proposed and existing accuracy measures
Our projected Auto associative neural network-based classification has categorized the discovered knowledge. The application of proposed method was applied by the Java platform. This projected work has attained the highest accuracy of the existing method. The accuracy value displays the ratio of support values we will attain better accuracy. And also, the projected work has taken less memory space and the minimum level of time taken to comprehensive the entire procedure. Henceforth, we can be demonstrated that our projected Auto associative neural network and relational suggestion rule mining method outdoes the state of work by accomplishing higher accuracy.
Author Contributions: Conceptualization, S.K., and D.G.; methodology, I.H.; software, I.H.; validation, I.H., S.S.A. and A.A.; formal analysis, I.H.; investigation, I.H., and S.S.A.; resources, I.H.; data curation, I.H.; writing—original draft preparation, S.K., and D.G.; writing—review and editing, S.K. and I.H.; visualization, S.K. and D.G.; supervision, D.G.; project administration, I.H., and S.S.A.; funding acquisition, I.H.; S.S.A. and A.A. All authors have read and agreed to the published version of the manuscript.
Acknowledgement: The authors are extremely thankful to Taif University for its financial support and furtherly the authors would like to extend their warm wishes to all anonymous reviewers and editors who supported us in making the work more worthy till its last stage.
Funding Statement: The authors are extremely thankful to Taif University for its esteemed support from Taif University Researchers supporting Project Number (TURSP-2020/215), Taif University, Taif, Saudi Arabia.
Conflicts of Interest: The authors declare that they have no conflicts of interest to report regarding the present study.
References
1. S. Suman, J. Petermann, T. Farrahi, A. Deshpande and G. C. Giakos, “Design, calibration and testing of an automated near-infrared liquid-crystal polarimetric imaging system for discrimination of lung cancer cells,” IEEE Transactions on Instrumentation and Measurement, vol. 64, no. 9, pp. 2453–2467, 2015. [Google Scholar]
2. P. Thomas, A. M. Barrutia and C. Ortiz-de-Solorzano, “A novel automated microscopy platform for multiresolution multispectral early detection of lung cancer cells in bronchoalveolar lavage samples,” IEEE Systems Journal, vol. 8, no. 3, pp. 985–994, 2013. [Google Scholar]
3. C. Paola, E. Casiraghi and D. Artioli, “A fully automated method for lung nodule detection from postero-anterior chest radiographs,” IEEE Transactions on Medical Imaging, vol. 25, no. 12, pp. 1588–1603, 2006. [Google Scholar]
4. K. Prashant, A. Druckman, J. Gallagher, B. Gatersleben, S. Allison et al., “The nexus between air pollution, green infrastructure and human health,” Environment International, vol. 133, pp. 105181, 2019. [Google Scholar]
5. G. Yuming, H. Zeng, R. Zheng, S. Li, A. G. Barnett et al., “The association between lung cancer incidence and ambient air pollution in China: A spatiotemporal analysis,” Environmental Research, vol. 144, pp. 60–65, 2016. [Google Scholar]
6. A. Imane, A. Verdin, F. Escande, F. S. Georges, F. Cazier et al., “In vitro short-term exposure to air pollution PM2. 5–0.3 induced cell cycle alterations and genetic instability in a human lung cell coculture model,” Environmental Research, vol. 147, pp. 146–158, 2016. [Google Scholar]
7. W. Liu, Z. Man, L. Hua, A. Chen, Y. Wang et al., “Data mining methods of lung cancer diagnosis by saliva tests using surface enhanced Raman spectroscopy,” in Proc. 7th Int. Conf. on Biomedical Engineering and Informatics, IEEE, Dalian, China, pp. 623–627, 2014, https://doi.org/10.1109/BMEI.2014.7002849. [Google Scholar]
8. I. Hussain, G. Samara, I. Ullah and N. Khan, “Encryption for end-user privacy: A cyber-secure smart energy management system,” in Proc. 22nd Int. Arab Conf. on Information Technology (ACIT), IEEE, Muscat, Oman, pp. 1–6, 2021, https://doi.org/10.1109/ACIT53391.2021.9677341. [Google Scholar]
9. G. Q. Gan, M. Koehoorn, H. W. Davies, P. A. Demers, L. Tamburic et al., “Long-term exposure to traffic-related air pollution and the risk of coronary heart disease hospitalization and mortality,” Environmental Health Perspectives, vol. 119, no. 4, pp. 501–507, 2011. [Google Scholar]
10. L. P. Mayala, M. M. Veiga and M. Babaei Khorzoughi, “Assessment of mine ventilation systems and air pollution impacts on artisanal tanzanite miners at merelani, Tanzania,” Journal of Cleaner Production, vol. 116, pp. 118–124, 2016. [Google Scholar]
11. X. Yang, X. Ye and G. Slabaugh, “Multilabel region classification and semantic linking for colon segmentation in CT colonography,” IEEE Transactions on Biomedical Engineering, vol. 62, no. 3, pp. 948–959, 2014. [Google Scholar]
12. Y. Takashi, S. Kashima, T. Tsuda, K. I. Takata, T. Ohta et al., “Long-term exposure to traffic-related air pollution and the risk of death from hemorrhagic stroke and lung cancer in shizuoka, Japan,” Science of the Total Environment, vol. 443, pp. 397–402, 2013. [Google Scholar]
13. T. Ruipeng, Y. Wang, X. Zhao and X. Yang, “Modeling health impacts of air pollutant emissions from the coal-fired power industry based on LCA and oriented by WTP: A case study,” Environmental Science and Pollution Research, vol. 29, no. 23, pp. 34486–34499, 2022. [Google Scholar]
14. I. Ullah, I. Hussain, K. Rehman, P. Wróblewski, W. Lewicki et al., “Exploiting the moth–flame optimization algorithm for optimal load management of the university campus: A viable approach in the academia sector,” Energies, vol. 15, no. 10, pp. 3741, 2022. https://doi.org/10.3390/en15103741. [Google Scholar]
15. R. Veerachamy, R. Ramalakshmi, B. P. Kavin, I. Hussain, A. H. Almaliki et al., “Exploiting IoT and its enabled technologies for irrigation needs in agriculture,” Water, vol. 14, no. 5, pp. 719, 2022. [Google Scholar]
16. S. J. Seung, M. Hurry, S. Hassan, R. N. Walton and W. K. Evans, “Cost-of-illness study for non-small-cell lung cancer using real-world data,” Current Oncology, vol. 26, no. 2, pp. 102–107, 2019. [Google Scholar]
17. W. Sun, G. C. Zhang, X. R. Zhang, X. Zhang and N. N. Ge, “Fine-grained vehicle type classification using lightweight convolutional neural network with feature optimization and joint learning strategy,” Multimedia Tools and Applications, vol. 80, no. 20, pp. 30803–30816, 2021. [Google Scholar]
18. W. Sun, X. Chen, X. R. Zhang, G. Z. Dai, P. S. Chang et al., “A Multi-feature learning model with enhanced local attention for vehicle re-identification,” Computers, Materials & Continua, vol. 69, no. 3, pp. 3549–3560, 2021. [Google Scholar]
19. M. Elnour, N. Meskin and M. Al-Naemi, “Sensor data validation and fault diagnosis using auto-associative neural network for HVAC systems,” Journal of Building Engineering, vol. 27, pp. 100935, 2020, ISSN 2352–7102. [Google Scholar]
20. I. Hussain, F. Khan, I. Ahmad, S. Khan and M. Saeed, “Power loss reduction via distributed generation system injected in a radial feeder,” Mehran University Research Journal of Engineering and Technology, vol. 40, no. 1, pp. 160–168, 2020, https://doi.org/10.22581/muet1982.2101.15. [Google Scholar]
21. I. Hussain, I. Ullah, W. Ali, G. Muhammad and Z. Ali, “Exploiting lion optimization algorithm for sustainable energy management system in industrial applications,” Sustainable Energy Technologies and Assessments, vol. 52, Part C, pp. 102237, 2022, ISSN 2213–1388, https://doi.org/10.1016/j.seta.2022.102237. [Google Scholar]
22. W. Ullah, I. Hussain, I. Shehzadi, Z. Rahman and P. Uthansakul, “Tracking a decentralized linear trajectory in an intermittent observation environment,” Sensors, vol. 20, no. 3, pp. 2127, 2020. https://doi.org/10.3390/s20072127. [Google Scholar]
23. I. Hussain, M. Ullah, I. Ullah, A. Bibi, M. Naeem et al., “Optimizing energy consumption in the home energy management system via a bio-inspired dragonfly algorithm and the genetic algorithm,” Electronics, vol. 9, no. 3, pp. 406, 2020. https://doi.org/10.3390/electronics9030406. [Google Scholar]
Cite This Article
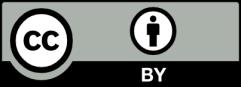
This work is licensed under a Creative Commons Attribution 4.0 International License , which permits unrestricted use, distribution, and reproduction in any medium, provided the original work is properly cited.