Open Access
ARTICLE
Smart Lung Tumor Prediction Using Dual Graph Convolutional Neural Network
Department of Computer Science, College of Arts and Sciences, Prince Sattam Bin Abdulaziz University, KSA
* Corresponding Author: Abdalla Alameen. Email:
Intelligent Automation & Soft Computing 2023, 36(1), 369-383. https://doi.org/10.32604/iasc.2023.031039
Received 08 April 2022; Accepted 21 June 2022; Issue published 29 September 2022
Abstract
A significant advantage of medical image processing is that it allows non-invasive exploration of internal anatomy in great detail. It is possible to create and study 3D models of anatomical structures to improve treatment outcomes, develop more effective medical devices, or arrive at a more accurate diagnosis. This paper aims to present a fused evolutionary algorithm that takes advantage of both whale optimization and bacterial foraging optimization to optimize feature extraction. The classification process was conducted with the aid of a convolutional neural network (CNN) with dual graphs. Evaluation of the performance of the fused model is carried out with various methods. In the initial input Computer Tomography (CT) image, 150 images are pre-processed and segmented to identify cancerous and non-cancerous nodules. The geometrical, statistical, structural, and texture features are extracted from the preprocessed segmented image using various methods such as Gray-level co-occurrence matrix (GLCM), Histogram-oriented gradient features (HOG), and Gray-level dependence matrix (GLDM). To select the optimal features, a novel fusion approach known as Whale-Bacterial Foraging Optimization is proposed. For the classification of lung cancer, dual graph convolutional neural networks have been employed. A comparison of classification algorithms and optimization algorithms has been conducted. According to the evaluated results, the proposed fused algorithm is successful with an accuracy of 98.72% in predicting lung tumors, and it outperforms other conventional approaches.Keywords
Cite This Article
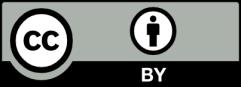
This work is licensed under a Creative Commons Attribution 4.0 International License , which permits unrestricted use, distribution, and reproduction in any medium, provided the original work is properly cited.