Open Access
ARTICLE
A Construction of Object Detection Model for Acute Myeloid Leukemia
1 Department of Computer Science and Engineering, Annamalai University, Chidambaram, 608002, India
2 School of Computer Science and Engineering, Vellore Institute of Technology, Vellore, 632014, India
* Corresponding Author: K. Venkatesh. Email:
Intelligent Automation & Soft Computing 2023, 36(1), 543-560. https://doi.org/10.32604/iasc.2023.030701
Received 31 March 2022; Accepted 21 June 2022; Issue published 29 September 2022
Abstract
The evolution of bone marrow morphology is necessary in Acute Myeloid Leukemia (AML) prediction. It takes an enormous number of times to analyze with the standardization and inter-observer variability. Here, we proposed a novel AML detection model using a Deep Convolutional Neural Network (D-CNN). The proposed Faster R-CNN (Faster Region-Based CNN) models are trained with Morphological Dataset. The proposed Faster R-CNN model is trained using the augmented dataset. For overcoming the Imbalanced Data problem, data augmentation techniques are imposed. The Faster R-CNN performance was compared with existing transfer learning techniques. The results show that the Faster R-CNN performance was significant than other techniques. The number of images in each class is different. For example, the Neutrophil (segmented) class consists of 8,486 images, and Lymphocyte (atypical) class consists of eleven images. The dataset is used to train the CNN for single-cell morphology classification. The proposed work implies the high-class performance server called Nvidia Tesla V100 GPU (Graphics processing unit).Keywords
Cite This Article
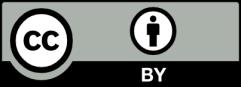
This work is licensed under a Creative Commons Attribution 4.0 International License , which permits unrestricted use, distribution, and reproduction in any medium, provided the original work is properly cited.