Open Access
ARTICLE
Generative Adversarial Networks for Secure Data Transmission in Wireless Network
Department of Computer Science, Government Arts College (A), Salem, 636007, Tamil Nadu, India
* Corresponding Author: E. Jayabalan. Email:
Intelligent Automation & Soft Computing 2023, 35(3), 3757-3784. https://doi.org/10.32604/iasc.2023.031200
Received 12 April 2022; Accepted 08 June 2022; Issue published 17 August 2022
Abstract
In this paper, a communication model in cognitive radios is developed and uses machine learning to learn the dynamics of jamming attacks in cognitive radios. It is designed further to make their transmission decision that automatically adapts to the transmission dynamics to mitigate the launched jamming attacks. The generative adversarial learning neural network (GALNN) or generative dynamic neural network (GDNN) automatically learns with the synthesized training data (training) with a generator and discriminator type neural networks that encompass minimax game theory. The elimination of the jamming attack is carried out with the assistance of the defense strategies and with an increased detection rate in the generative adversarial network (GAN). The GDNN with game theory is designed to validate the channel condition with the cross entropy loss function and back-propagation algorithm, which improves the communication reliability in the network. The simulation is conducted in NS2.34 tool against several performance metrics to reduce the misdetection rate and false alarm rates. The results show that the GDNN obtains an increased rate of successful transmission by taking optimal actions to act as a defense mechanism to mislead the jammer, where the jammer makes high misclassification errors on transmission dynamics.Keywords
Cite This Article
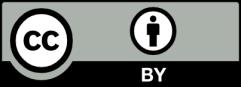
This work is licensed under a Creative Commons Attribution 4.0 International License , which permits unrestricted use, distribution, and reproduction in any medium, provided the original work is properly cited.