Open Access
ARTICLE
Eye Strain Detection During Online Learning
1 Faculty of Physics, VNU University of Science, Hanoi, 100000, Vietnam
2 University of Science, Vietnam National University, Hanoi, 100000, Vietnam
3 VNU-HUS, High School for Gifted Students, Hanoi, 100000, Vietnam
4 Hanoi-Amsterdam High School for the Gifted, Hanoi, 100000, Vietnam
* Corresponding Author: Le Quang Thao. Email:
Intelligent Automation & Soft Computing 2023, 35(3), 3517-3530. https://doi.org/10.32604/iasc.2023.031026
Received 08 April 2022; Accepted 08 June 2022; Issue published 17 August 2022
Abstract
The recent outbreak of the coronavirus disease of 2019 (Covid-19) has been causing many disruptions among the education systems worldwide, most of them due to the abrupt transition to online learning. The sudden upsurge in digital electronic devices usage, namely personal computers, laptops, tablets and smartphones is unprecedented, which leads to a new wave of both mental and physical health problems among students, for example eye-related illnesses. The overexposure to electronic devices, extended screen time usage and lack of outdoor sunlight have put a consequential strain on the student’s ophthalmic health because of their young age and a relative lack of responsibility on their own health. Failure to take appropriate external measures to mitigate the negative effects of this process could lead to common ophthalmic illnesses such as myopia or more serious conditions. To remedy this situation, we propose a software solution that is able to track and capture images of its users’ eyes to detect symptoms of eye illnesses while simultaneously giving them warnings and even offering treatments. To meet the requirements of a small and light model that is operable on low-end devices without information loss, we optimized the original MobileNetV2 model with depth-wise separable convolutions by altering the parameters in the last layers with an aim to minimize the resizing of the input image and obtained a new model which we call EyeNet. Combined with applying the knowledge distillation technique and ResNet-18 as a teacher model to train the student model, we have successfully increased the accuracy of the EyeNet model up to 87.16% and support the development of a model compatible with embedded systems with limited computing power, accessible to all students.Keywords
Cite This Article
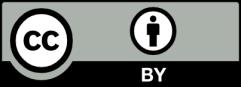