Open Access
ARTICLE
Encephalitis Detection from EEG Fuzzy Density-Based Clustering Model with Multiple Centroid
1 Department of Information Systems, College of Computer and Information Sciences, Princess Nourah Bint Abdulrahman University, Riyadh, 11671, Saudi Arabia
2 Department of Information Systems, College of Computer and Information Sciences, King Saud University, Riyadh, Saudi Arabia
3 Department of Computer Sciences, College of Computer and Information Sciences, Princess Nourah Bint Abdulrahman University, P.O. Box 84428, Riyadh 11671, Saudi Arabia
* Corresponding Author: Hanan A. Hosni Mahmoud. Email:
Intelligent Automation & Soft Computing 2023, 35(3), 3129-3140. https://doi.org/10.32604/iasc.2023.030836
Received 03 April 2022; Accepted 17 May 2022; Issue published 17 August 2022
Abstract
Encephalitis is a brain inflammation disease. Encephalitis can yield to seizures, motor disability, or some loss of vision or hearing. Sometimes, encephalitis can be a life-threatening and proper diagnosis in an early stage is very crucial. Therefore, in this paper, we are proposing a deep learning model for computerized detection of Encephalitis from the electroencephalogram data (EEG). Also, we propose a Density-Based Clustering model to classify the distinctive waves of Encephalitis. Customary clustering models usually employ a computed single centroid virtual point to define the cluster configuration, but this single point does not contain adequate information. To precisely extract accurate inner structural data, a multiple centroids approach is employed and defined in this paper, which defines the cluster configuration by allocating weights to each state in the cluster. The multiple EEG view fuzzy learning approach incorporates data from every single view to enhance the model's clustering performance. Also a fuzzy Density-Based Clustering model with multiple centroids (FDBC) is presented. This model employs multiple real state centroids to define clusters using Partitioning Around Centroids algorithm. The Experimental results validate the medical importance of the proposed clustering model.Keywords
Cite This Article
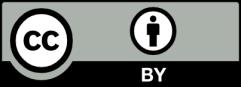