Open Access
ARTICLE
Automatic Clustering of User Behaviour Profiles for Web Recommendation System
1 Department of Computer Science and Engineering, Velalar College of Engineering and Technology, Erode, 638 012, Tamil Nadu, India
2 Al-Nahrain Nanorenewable Energy Research Center, Al-Nahrain University, Baghdad, 64074, Iraq
3 Department of Computer Science, College of Computers and Information Technology, Taif University, P.O. Box 11099, Taif 21944, Saudi Arabia
4 Department of Computer Science and Engineering, Kongu Engineering College, Perundurai, 638060, Tamil Nadu, India
5 School of Digital Science, University Brunei Darussalam, Jln Tungku Link, Gadong BE1410, Brunei Darussalam
* Corresponding Author: S. Sadesh. Email:
Intelligent Automation & Soft Computing 2023, 35(3), 3365-3384. https://doi.org/10.32604/iasc.2023.030751
Received 01 April 2022; Accepted 26 May 2022; Issue published 17 August 2022
Abstract
Web usage mining, content mining, and structure mining comprise the web mining process. Web-Page Recommendation (WPR) development by incorporating Data Mining Techniques (DMT) did not include end-users with improved performance in the obtained filtering results. The cluster user profile-based clustering process is delayed when it has a low precision rate. Markov Chain Monte Carlo-Dynamic Clustering (MC2-DC) is based on the User Behavior Profile (UBP) model group’s similar user behavior on a dynamic update of UBP. The Reversible-Jump Concept (RJC) reviews the history with updated UBP and moves to appropriate clusters. Hamilton’s Filtering Framework (HFF) is designed to filter user data based on personalised information on automatically updated UBP through the Search Engine (SE). The Hamilton Filtered Regime Switching User Query Probability (HFRSUQP) works forward the updated UBP for easy and accurate filtering of users’ interests and improves WPR. A Probabilistic User Result Feature Ranking based on Gaussian Distribution (PURFR-GD) has been developed to user rank results in a web mining process. PURFR-GD decreases the delay time in the end-to-end workflow for SE personalization in various methods by using the Gaussian Distribution Function (GDF). The theoretical analysis and experiment results of the proposed MC2-DC method automatically increase the updated UBP accuracy by 18.78%. HFRSUQP enabled extensive Maximize Log-Likelihood (ML-L) increases to 15.28% of User Personalized Information Search Retrieval Rate (UPISRT). For feature ranking, the PURFR-GD model defines higher Classification Accuracy (CA) and Precision Ratio (PR) while utilising minimum Execution Time (ET). Furthermore, UPISRT's ranking performance has improved by 20%.Keywords
Cite This Article
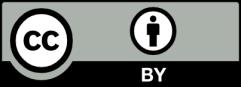
This work is licensed under a Creative Commons Attribution 4.0 International License , which permits unrestricted use, distribution, and reproduction in any medium, provided the original work is properly cited.