Open Access
ARTICLE
Arithmetic Optimization with Deep Learning Enabled Churn Prediction Model for Telecommunication Industries
SSN School of Management, Kalavakkam, Chennai, 603110, India
* Corresponding Author: Vani Haridasan. Email:
Intelligent Automation & Soft Computing 2023, 35(3), 3531-3544. https://doi.org/10.32604/iasc.2023.030628
Received 30 March 2022; Accepted 08 June 2022; Issue published 17 August 2022
Abstract
Customer retention is one of the challenging issues in different business sectors, and various firms utilize customer churn prediction (CCP) process to retain existing customers. Because of the direct impact on the company revenues, particularly in the telecommunication sector, firms are needed to design effective CCP models. The recent advances in machine learning (ML) and deep learning (DL) models enable researchers to introduce accurate CCP models in the telecommunication sector. CCP can be considered as a classification problem, which aims to classify the customer into churners and non-churners. With this motivation, this article focuses on designing an arithmetic optimization algorithm (AOA) with stacked bidirectional long short-term memory (SBLSTM) model for CCP. The proposed AOA-SBLSTM model intends to proficiently forecast the occurrence of CC in the telecommunication industry. Initially, the AOA-SBLSTM model performs pre-processing to transform the original data into a useful format. Besides, the SBLSTM model is employed to categorize data into churners and non-churners. To improve the CCP outcomes of the SBLSTM model, an optimal hyperparameter tuning process using AOA is developed. A widespread simulation analysis of the AOA-SBLSTM model is tested using a benchmark dataset with 3333 samples and 21 features. The experimental outcomes reported the promising performance of the AOA-SBLSTM model over the recent approaches.Keywords
Cite This Article
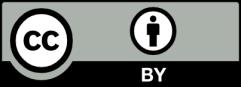
This work is licensed under a Creative Commons Attribution 4.0 International License , which permits unrestricted use, distribution, and reproduction in any medium, provided the original work is properly cited.