Open Access
ARTICLE
Deep Learning for Wind Speed Forecasting Using Bi-LSTM with Selected Features
1 Department of Computer Science and Engineering, Sreenivasa Institute of Technology and Management Studies, Chittoor, 517127, India
2 School of Information Technology & Engineering, Vellore Institute of Technology, Vellore, 632014, India
3 School of Computer Science and Engineering, Vellore Institute of Technology, Chennai, 600127, India
4 School of Computing, College of Engineering and Technology, SRM Institute of Science and Technology, Kattankulathur, 603203, India
* Corresponding Author: Senthil Kumar Paramasivan. Email:
Intelligent Automation & Soft Computing 2023, 35(3), 3829-3844. https://doi.org/10.32604/iasc.2023.030480
Received 27 March 2022; Accepted 25 May 2022; Issue published 17 August 2022
Abstract
Wind speed forecasting is important for wind energy forecasting. In the modern era, the increase in energy demand can be managed effectively by forecasting the wind speed accurately. The main objective of this research is to improve the performance of wind speed forecasting by handling uncertainty, the curse of dimensionality, overfitting and non-linearity issues. The curse of dimensionality and overfitting issues are handled by using Boruta feature selection. The uncertainty and the non-linearity issues are addressed by using the deep learning based Bi-directional Long Short Term Memory (Bi-LSTM). In this paper, Bi-LSTM with Boruta feature selection named BFS-Bi-LSTM is proposed to improve the performance of wind speed forecasting. The model identifies relevant features for wind speed forecasting from the meteorological features using Boruta wrapper feature selection (BFS). Followed by Bi-LSTM predicts the wind speed by considering the wind speed from the past and future time steps. The proposed BFS-Bi-LSTM model is compared against Multilayer perceptron (MLP), MLP with Boruta (BFS-MLP), Long Short Term Memory (LSTM), LSTM with Boruta (BFS-LSTM) and Bi-LSTM in terms of Root Mean Square Error (RMSE), Mean Absolute Error (MAE), Mean Square Error (MSE) and R2. The BFS-Bi-LSTM surpassed other models by producing RMSE of 0.784, MAE of 0.530, MSE of 0.615 and R2 of 0.8766. The experimental result shows that the BFS-Bi-LSTM produced better forecasting results compared to others.Keywords
Cite This Article
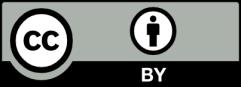