Open Access
ARTICLE
Brain Tumor Classification Using Image Fusion and EFPA-SVM Classifier
1 Anna University, Chennai, 600025, India
2 Department of Computer Science and Engineering, Tamilnadu College of Engineering, Coimbatore, 641659, India
* Corresponding Author: P. P. Fathimathul Rajeena. Email:
Intelligent Automation & Soft Computing 2023, 35(3), 2837-2855. https://doi.org/10.32604/iasc.2023.030144
Received 19 March 2022; Accepted 03 May 2022; Issue published 17 August 2022
Abstract
An accurate and early diagnosis of brain tumors based on medical imaging modalities is of great interest because brain tumors are a harmful threat to a person’s health worldwide. Several medical imaging techniques have been used to analyze brain tumors, including computed tomography (CT) and magnetic resonance imaging (MRI). CT provides information about dense tissues, whereas MRI gives information about soft tissues. However, the fusion of CT and MRI images has little effect on enhancing the accuracy of the diagnosis of brain tumors. Therefore, machine learning methods have been adopted to diagnose brain tumors in recent years. This paper intends to develop a novel scheme to detect and classify brain tumors based on fused CT and MRI images. The proposed approach starts with preprocessing the images to reduce the noise. Then, fusion rules are applied to get the fused image, and a segmentation algorithm is employed to isolate the tumor region from the background to isolate the tumor region. Finally, a machine learning classifier classified the brain images into benign and malignant tumors. Computing statistical measures evaluate the classification potential of the proposed scheme. Experimental outcomes are provided, and the Enhanced Flower Pollination Algorithm (EFPA) system shows that it outperforms other brain tumor classification methods considered for comparison.Keywords
Cite This Article
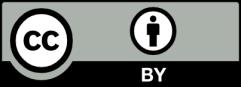
This work is licensed under a Creative Commons Attribution 4.0 International License , which permits unrestricted use, distribution, and reproduction in any medium, provided the original work is properly cited.