Open Access
ARTICLE
Unconstrained Gender Recognition from Periocular Region Using Multiscale Deep Features
Department of Computer Science, College of Computer and Information Sciences, King Saud University, Riyadh, 11451, Saudi Arabia
* Corresponding Author: Muhammad Hussain. Email:
Intelligent Automation & Soft Computing 2023, 35(3), 2941-2962. https://doi.org/10.32604/iasc.2023.030036
Received 17 March 2022; Accepted 05 May 2022; Issue published 17 August 2022
Abstract
The gender recognition problem has attracted the attention of the computer vision community due to its importance in many applications (e.g., surveillance and human–computer interaction [HCI]). Images of varying levels of illumination, occlusion, and other factors are captured in uncontrolled environments. Iris and facial recognition technology cannot be used on these images because iris texture is unclear in these instances, and faces may be covered by a scarf, hijab, or mask due to the COVID-19 pandemic. The periocular region is a reliable source of information because it features rich discriminative biometric features. However, most existing gender classification approaches have been designed based on hand-engineered features or validated in controlled environments. Motivated by the superior performance of deep learning, we proposed a new method, PeriGender, inspired by the design principles of the ResNet and DenseNet models, that can classify gender using features from the periocular region. The proposed system utilizes a dense concept in a residual model. Through skip connections, it reuses features on different scales to strengthen discriminative features. Evaluations of the proposed system on challenging datasets indicated that it outperformed state-of-the-art methods. It achieved 87.37%, 94.90%, 94.14%, 99.14%, and 95.17% accuracy on the GROUPS, UFPR-Periocular, Ethnic-Ocular, IMP, and UBIPr datasets, respectively, in the open-world (OW) protocol. It further achieved 97.57% and 93.20% accuracy for adult periocular images from the GROUPS dataset in the closed-world (CW) and OW protocols, respectively. The results showed that the middle region between the eyes plays a crucial role in the recognition of masculine features, and feminine features can be identified through the eyebrow, upper eyelids, and corners of the eyes. Furthermore, using a whole region without cropping enhances PeriGender’s learning capability, improving its understanding of both eyes’ global structure without discontinuity.Keywords
Cite This Article
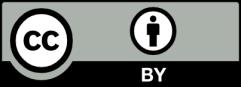