Open Access
ARTICLE
Cephalopods Classification Using Fine Tuned Lightweight Transfer Learning Models
1 Department of Computer Science & Engineering, Sri Krishna College of Technology, Coimbatore, 641042, Tamil Nadu, India
2 Department of Electronics and Communication Engineering, Government College of Technology, Coimbatore, 641013, Tamil Nadu, India
3 Department of Marine Pharmacology, Faculty of Allied Health Sciences, Chettinad Academy of Research and Education, Kelambakkam, Chengalpattu, 603103, Tamil Nadu, India
* Corresponding Author: P. Anantha Prabha. Email:
Intelligent Automation & Soft Computing 2023, 35(3), 3065-3079. https://doi.org/10.32604/iasc.2023.030017
Received 16 March 2022; Accepted 26 April 2022; Issue published 17 August 2022
Abstract
Cephalopods identification is a formidable task that involves hand inspection and close observation by a malacologist. Manual observation and identification take time and are always contingent on the involvement of experts. A system is proposed to alleviate this challenge that uses transfer learning techniques to classify the cephalopods automatically. In the proposed method, only the Lightweight pre-trained networks are chosen to enable IoT in the task of cephalopod recognition. First, the efficiency of the chosen models is determined by evaluating their performance and comparing the findings. Second, the models are fine-tuned by adding dense layers and tweaking hyperparameters to improve the classification of accuracy. The models also employ a well-tuned Rectified Adam optimizer to increase the accuracy rates. Third, Adam with Gradient Centralisation (RAdamGC) is proposed and used in fine-tuned models to reduce the training time. The framework enables an Internet of Things (IoT) or embedded device to perform the classification tasks by embedding a suitable lightweight pre-trained network. The fine-tuned models, MobileNetV2, InceptionV3, and NASNet Mobile have achieved a classification accuracy of 89.74%, 87.12%, and 89.74%, respectively. The findings have indicated that the fine-tuned models can classify different kinds of cephalopods. The results have also demonstrated that there is a significant reduction in the training time with RAdamGC.Keywords
Cite This Article
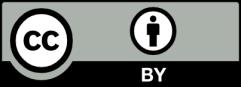
This work is licensed under a Creative Commons Attribution 4.0 International License , which permits unrestricted use, distribution, and reproduction in any medium, provided the original work is properly cited.