Open Access
ARTICLE
Optimal Deep Belief Network Enabled Malware Detection and Classification Model
1 Department of Computer and Information Science, Faculty of Science, Annamalai University, Chidambaram, 608002, India
2 Department of CSE, Alagappa Chettiar Government College of Engineering and Technology, Karaikudi, 630003, India
3 Department of Computer and Information Science, Annamalai University, Chidambaram, 608002, India
* Corresponding Author: P. Pandi Chandran. Email:
Intelligent Automation & Soft Computing 2023, 35(3), 3349-3364. https://doi.org/10.32604/iasc.2023.029946
Received 15 March 2022; Accepted 20 April 2022; Issue published 17 August 2022
Abstract
Cybercrime has increased considerably in recent times by creating new methods of stealing, changing, and destroying data in daily lives. Portable Document Format (PDF) has been traditionally utilized as a popular way of spreading malware. The recent advances of machine learning (ML) and deep learning (DL) models are utilized to detect and classify malware. With this motivation, this study focuses on the design of mayfly optimization with a deep belief network for PDF malware detection and classification (MFODBN-MDC) technique. The major intention of the MFODBN-MDC technique is for identifying and classifying the presence of malware exist in the PDFs. The proposed MFODBN-MDC method derives a new MFO algorithm for the optimal selection of feature subsets. In addition, Adamax optimizer with the DBN model is used for PDF malware detection and classification. The design of the MFO algorithm to select features and Adamax based hyperparameter tuning for PDF malware detection and classification demonstrates the novelty of the work. For demonstrating the improved outcomes of the MFODBN-MDC model, a wide range of simulations are executed, and the results are assessed in various aspects. The comparison study highlighted the enhanced outcomes of the MFODBN-MDC model over the existing techniques with maximum precision, recall, and F1 score of 97.42%, 97.33%, and 97.33%, respectively.Keywords
Cite This Article
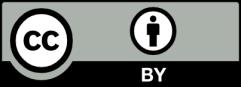