Open Access
ARTICLE
Recent Advances in Fatigue Detection Algorithm Based on EEG
1 School of Software, South China Normal University, FoShan, 528225, China
2 Pazhou Lab, Guangzhou, 510330, China
3 Department of Psychiatry, New York University School of Medicine, New York, 10016, USA
* Corresponding Author: Jingcong Li. Email:
Intelligent Automation & Soft Computing 2023, 35(3), 3573-3586. https://doi.org/10.32604/iasc.2023.029698
Received 09 March 2022; Accepted 14 April 2022; Issue published 17 August 2022
Abstract
Fatigue is a state commonly caused by overworked, which seriously affects daily work and life. How to detect mental fatigue has always been a hot spot for researchers to explore. Electroencephalogram (EEG) is considered one of the most accurate and objective indicators. This article investigated the development of classification algorithms applied in EEG-based fatigue detection in recent years. According to the different source of the data, we can divide these classification algorithms into two categories, intra-subject (within the same subject) and cross-subject (across different subjects). In most studies, traditional machine learning algorithms with artificial feature extraction methods were commonly used for fatigue detection as intra-subject algorithms. Besides, deep learning algorithms have been applied to fatigue detection and could achieve effective result based on large-scale dataset. However, it is difficult to perform long-term calibration training on the subjects in practical applications. With the lack of large samples, transfer learning algorithms as a cross-subject algorithm could promote the practical application of fatigue detection methods. We found that the research based on deep learning and transfer learning has gradually increased in recent years. But as a field with increasing requirements, researchers still need to continue to explore efficient decoding algorithms, design effective experimental paradigms, and collect and accumulate valid standard data, to achieve fast and accurate fatigue detection methods or systems to further widely apply.Keywords
Cite This Article
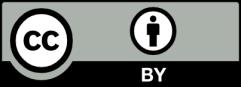
This work is licensed under a Creative Commons Attribution 4.0 International License , which permits unrestricted use, distribution, and reproduction in any medium, provided the original work is properly cited.