Open Access
ARTICLE
A Novel Approach to Design Distribution Preserving Framework for Big Data
1 Department of Information Technology, St. Peter’s College of Engineering and Technology, Chennai, 600054, Tamilnadu, India
2 Department of Information Technology, R.M.D Engineering College, Chennai, 601206, Tamilnadu, India
* Corresponding Author: Mini Prince. Email:
Intelligent Automation & Soft Computing 2023, 35(3), 2789-2803. https://doi.org/10.32604/iasc.2023.029533
Received 05 March 2022; Accepted 13 April 2022; Issue published 17 August 2022
Abstract
In several fields like financial dealing, industry, business, medicine, et cetera, Big Data (BD) has been utilized extensively, which is nothing but a collection of a huge amount of data. However, it is highly complicated along with time-consuming to process a massive amount of data. Thus, to design the Distribution Preserving Framework for BD, a novel methodology has been proposed utilizing Manhattan Distance (MD)-centered Partition Around Medoid (MD–PAM) along with Conjugate Gradient Artificial Neural Network (CG-ANN), which undergoes various steps to reduce the complications of BD. Firstly, the data are processed in the pre-processing phase by mitigating the data repetition utilizing the map-reduce function; subsequently, the missing data are handled by substituting or by ignoring the missed values. After that, the data are transmuted into a normalized form. Next, to enhance the classification performance, the data’s dimensionalities are minimized by employing Gaussian Kernel (GK)-Fisher Discriminant Analysis (GK-FDA). Afterwards, the processed data is submitted to the partitioning phase after transmuting it into a structured format. In the partition phase, by utilizing the MD-PAM, the data are partitioned along with grouped into a cluster. Lastly, by employing CG-ANN, the data are classified in the classification phase so that the needed data can be effortlessly retrieved by the user. To analogize the outcomes of the CG-ANN with the prevailing methodologies, the NSL-KDD openly accessible datasets are utilized. The experiential outcomes displayed that an efficient result along with a reduced computation cost was shown by the proposed CG-ANN. The proposed work outperforms well in terms of accuracy, sensitivity and specificity than the existing systems.
Keywords
Cite This Article
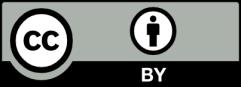
This work is licensed under a Creative Commons Attribution 4.0 International License , which permits unrestricted use, distribution, and reproduction in any medium, provided the original work is properly cited.