Open Access
ARTICLE
Robust Deep Transfer Learning Based Object Detection and Tracking Approach
1 Electronics and Communication Engineering, Periyar Maniammai Institute of Science & Technology(PMIST), Thanjavur, 613403, India
2 Department of Computer Science and Engineering, Periyar Maniammai Institute of Science & Technology, Thanjavur, 613403, India
3 Department of Electrical and Electronics Engineering, Periyar Maniammai Institute of Science and Technology, Thanjavur, 613403, India
4 Electrical Engineering Department, Model Institute of Engineering & Technology (Autonomous), Jammu, 181122, India
5 Department of Computer Science and Engineering, Shri Mata Vaishno Devi University, Katra, 182320, India
6 Center for Energy Research, Chennai Institute of Technology, Chennai, 600069, India
* Corresponding Author: B. Jegajothi. Email:
Intelligent Automation & Soft Computing 2023, 35(3), 3613-3626. https://doi.org/10.32604/iasc.2023.029323
Received 01 March 2022; Accepted 25 May 2022; Issue published 17 August 2022
Abstract
At present days, object detection and tracking concepts have gained more importance among researchers and business people. Presently, deep learning (DL) approaches have been used for object tracking as it increases the performance and speed of the tracking process. This paper presents a novel robust DL based object detection and tracking algorithm using Automated Image Annotation with ResNet based Faster regional convolutional neural network (R-CNN) named (AIA-FRCNN) model. The AIA-RFRCNN method performs image annotation using a Discriminative Correlation Filter (DCF) with Channel and Spatial Reliability tracker (CSR) called DCF-CSRT model. The AIA-RFRCNN model makes use of Faster RCNN as an object detector and tracker, which involves region proposal network (RPN) and Fast R-CNN. The RPN is a full convolution network that concurrently predicts the bounding box and score of different objects. The RPN is a trained model used for the generation of the high-quality region proposals, which are utilized by Fast R-CNN for detection process. Besides, Residual Network (ResNet 101) model is used as a shared convolutional neural network (CNN) for the generation of feature maps. The performance of the ResNet 101 model is further improved by the use of Adam optimizer, which tunes the hyperparameters namely learning rate, batch size, momentum, and weight decay. Finally, softmax layer is applied to classify the images. The performance of the AIA-RFRCNN method has been assessed using a benchmark dataset and a detailed comparative analysis of the results takes place. The outcome of the experiments indicated the superior characteristics of the AIA-RFRCNN model under diverse aspects.Keywords
Cite This Article
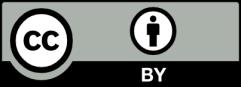