Open Access
ARTICLE
Energy Efficient Networks Using Ant Colony Optimization with Game Theory Clustering
1 Department of Computer Science and Engineering, Hosur, 635117, India
2 Er. Perumal Manimekalai College of Engineering, Hosur, 635117, India
* Corresponding Author: Harish Gunigari. Email:
Intelligent Automation & Soft Computing 2023, 35(3), 3557-3571. https://doi.org/10.32604/iasc.2023.029155
Received 26 February 2022; Accepted 19 April 2022; Issue published 17 August 2022
Abstract
Real-time applications based on Wireless Sensor Network (WSN) technologies quickly lead to the growth of an intelligent environment. Sensor nodes play an essential role in distributing information from networking and its transfer to the sinks. The ability of dynamical technologies and related techniques to be aided by data collection and analysis across the Internet of Things (IoT) network is widely recognized. Sensor nodes are low-power devices with low power devices, storage, and quantitative processing capabilities. The existing system uses the Artificial Immune System-Particle Swarm Optimization method to minimize the energy and improve the network’s lifespan. In the proposed system, a hybrid Energy Efficient and Reliable Ant Colony Optimization (ACO) based on the Routing protocol (E-RARP) and game theory-based energy-efficient clustering algorithm (GEC) were used. E-RARP is a new Energy Efficient, and Reliable ACO-based Routing Protocol for Wireless Sensor Networks. The suggested protocol provides communications dependability and high-quality channels of communication to improve energy. For wireless sensor networks, a game theory-based energy-efficient clustering technique (GEC) is used, in which each sensor node is treated as a player on the team. The sensor node can choose beneficial methods for itself, determined by the length of idle playback time in the active phase, and then decide whether or not to rest. The proposed E-RARP-GEC improves the network’s lifetime and data transmission; it also takes a minimum amount of energy compared with the existing algorithms.Keywords
Cite This Article
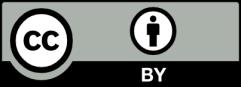
This work is licensed under a Creative Commons Attribution 4.0 International License , which permits unrestricted use, distribution, and reproduction in any medium, provided the original work is properly cited.