Open Access
ARTICLE
LSTM Based Spectrum Prediction for Real-Time Spectrum Access for IoT Applications
1 KSR Institute for Engineering and Technology, Tiruchencode, 637215, India
2 ECE Department, SRMIST, Chengalpattu, Chennai, 603202, India
* Corresponding Author: Vijayakumar Ponnusamy. Email:
Intelligent Automation & Soft Computing 2023, 35(3), 2805-2819. https://doi.org/10.32604/iasc.2023.028645
Received 14 February 2022; Accepted 15 April 2022; Issue published 17 August 2022
Abstract
In the Internet of Things (IoT) scenario, many devices will communicate in the presence of the cellular network; the chances of availability of spectrum will be very scary given the presence of large numbers of mobile users and large amounts of applications. Spectrum prediction is very encouraging for high traffic next-generation wireless networks, where devices/machines which are part of the Cognitive Radio Network (CRN) can predict the spectrum state prior to transmission to save their limited energy by avoiding unnecessarily sensing radio spectrum. Long short-term memory (LSTM) is employed to simultaneously predict the Radio Spectrum State (RSS) for two-time slots, thereby allowing the secondary node to use the prediction result to transmit its information to achieve lower waiting time hence, enhanced performance capacity. A framework of spectral transmission based on the LSTM prediction is formulated, named as positive prediction and sensing-based spectrum access. The proposed scheme provides an average maximum waiting time gain of 2.88 ms. The proposed scheme provides 0.096 bps more capacity than a conventional energy detector.Keywords
Cite This Article
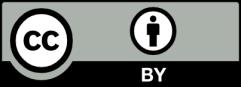
This work is licensed under a Creative Commons Attribution 4.0 International License , which permits unrestricted use, distribution, and reproduction in any medium, provided the original work is properly cited.