Open Access
ARTICLE
A Graph Theory Based Self-Learning Honeypot to Detect Persistent Threats
1 Department of Mathematics, College of Engineering, Anna University, Chennai, 600 025, India
2 Department of Networking and Communications, School of Computing, SRM Institute of Science and Technology, Kattankulathur, 602 203, India
* Corresponding Author: R. T. Pavendan. Email:
Intelligent Automation & Soft Computing 2023, 35(3), 3331-3348. https://doi.org/10.32604/iasc.2023.028029
Received 31 January 2022; Accepted 15 March 2022; Issue published 17 August 2022
Abstract
Attacks on the cyber space is getting exponential in recent times. Illegal penetrations and breaches are real threats to the individuals and organizations. Conventional security systems are good enough to detect the known threats but when it comes to Advanced Persistent Threats (APTs) they fails. These APTs are targeted, more sophisticated and very persistent and incorporates lot of evasive techniques to bypass the existing defenses. Hence, there is a need for an effective defense system that can achieve a complete reliance of security. To address the above-mentioned issues, this paper proposes a novel honeypot system that tracks the anonymous behavior of the APT threats. The key idea of honeypot leverages the concepts of graph theory to detect such targeted attacks. The proposed honeypot is self-realizing, strategic assisted which withholds the APTs actionable techniques and observes the behavior for analysis and modelling. The proposed graph theory based self learning honeypot using the results γ(C(n,1)),γc (C(n,1)), γsc (C(n,1)) outperforms traditional techniques by detecting APTs behavioral with detection rate of 96%.Keywords
Cite This Article
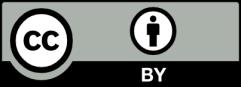
This work is licensed under a Creative Commons Attribution 4.0 International License , which permits unrestricted use, distribution, and reproduction in any medium, provided the original work is properly cited.