Open Access
ARTICLE
Automatic Anomaly Monitoring in Public Surveillance Areas
1 Department of Electrical Engineering, College of Engineering, King Faisal University, Al-Ahsa, 31982, Saudi Arabia
2 Department of Computer Science, Bahria University, Islamabad, Pakistan
3 Department of Computer Science and Software Engineering, Al Ain University, Al Ain, 15551, UAE
4 Department of Humanities and Social Science, Al Ain University, Al Ain, 15551, UAE
5 Department of Computer Science, College of Computer, Qassim University, Buraydah, 51452, Saudi Arabia
6 Department of Computer Science, Air University, Islamabad, Pakistan
7 Department of Computer Engineering, Korea Polytechnic University, 237 Sangidaehak-ro Siheung-si, Gyeonggi-do, 15073, Korea
* Corresponding Author: Jeongmin Park. Email:
Intelligent Automation & Soft Computing 2023, 35(3), 2655-2671. https://doi.org/10.32604/iasc.2023.027205
Received 13 January 2022; Accepted 08 June 2022; Issue published 17 August 2022
Abstract
With the dramatic increase in video surveillance applications and public safety measures, the need for an accurate and effective system for abnormal/suspicious activity classification also increases. Although it has multiple applications, the problem is very challenging. In this paper, a novel approach for detecting normal/abnormal activity has been proposed. We used the Gaussian Mixture Model (GMM) and Kalman filter to detect and track the objects, respectively. After that, we performed shadow removal to segment an object and its shadow. After object segmentation we performed occlusion detection method to detect occlusion between multiple human silhouettes and we implemented a novel method for region shrinking to isolate occluded humans. Fuzzy c-mean is utilized to verify human silhouettes and motion based features including velocity and optical flow are extracted for each identified silhouettes. Gray Wolf Optimizer (GWO) is used to optimize feature set followed by abnormal event classification that is performed using the XG-Boost classifier. This system is applicable in any surveillance application used for event detection or anomaly detection. Performance of proposed system is evaluated using University of Minnesota (UMN) dataset and UBI (University of Beira Interior)-Fight dataset, each having different type of anomaly. The mean accuracy for the UMN and UBI-Fight datasets is 90.14% and 76.9% respectively. These results are more accurate as compared to other existing methods.Keywords
Cite This Article
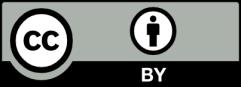