Open Access
ARTICLE
Forecasting Stock Volatility Using Wavelet-based Exponential Generalized Autoregressive Conditional Heteroscedasticity Methods
1 School of Mathematical Science, Universiti Sains Malaysia, Penang, Malaysia
2 Department of Risk Management and Insurance, Faculty of Business, The University of Jordan, Jordan
3 Department of Basic Sciences, College of Science and Theoretical Studies, Saudi Electronic University, Riyadh, Saudi Arabia
* Corresponding Author: Nawaf N. Hamadneh. Email:
Intelligent Automation & Soft Computing 2023, 35(3), 2589-2601. https://doi.org/10.32604/iasc.2023.024001
Received 30 September 2021; Accepted 11 February 2022; Issue published 17 August 2022
Abstract
In this study, we proposed a new model to improve the accuracy of forecasting the stock market volatility pattern. The hypothesized model was validated empirically using a data set collected from the Saudi Arabia stock Exchange (Tadawul). The data is the daily closed price index data from August 2011 to December 2019 with 2027 observations. The proposed forecasting model combines the best maximum overlapping discrete wavelet transform (MODWT) function (Bl14) and exponential generalized autoregressive conditional heteroscedasticity (EGARCH) model. The results show the model's ability to analyze stock market data, highlight important events that contain the most volatile data, and improve forecast accuracy. The results were compared from a number of mathematical models, which are the non-linear spectral model, autoregressive integrated moving average (ARIMA) model and EGARCH model. The performance of the forecasting model will be evaluated based on some of error functions such as Mean absolute percentage error (MAPE), Mean absolute scaled error (MASE) and Root means squared error (RMSE).Keywords
Cite This Article
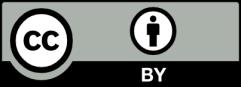