Open Access
ARTICLE
Investigation of Android Malware Using Deep Learning Approach
1 Department of Computer Science and Engineering, Sathyabama Institute of Science and Technology, Chennai, 600119, Tamilnadu, India
2 School of Computing, SRM Institute of Science and Technology, Chennai, 603203, Tamilnadu, India
* Corresponding Author: V. Joseph Raymond. Email:
Intelligent Automation & Soft Computing 2023, 35(2), 2413-2429. https://doi.org/10.32604/iasc.2023.030527
Received 28 March 2022; Accepted 05 May 2022; Issue published 19 July 2022
Abstract
In recent days the usage of android smartphones has increased extensively by end-users. There are several applications in different categories banking/finance, social engineering, education, sports and fitness, and many more applications. The android stack is more vulnerable compared to other mobile platforms like IOS, Windows, or Blackberry because of the open-source platform. In the Existing system, malware is written using vulnerable system calls to bypass signature detection important drawback is might not work with zero-day exploits and stealth malware. The attackers target the victim with various attacks like adware, backdoor, spyware, ransomware, and zero-day exploits and create threat hunts on the day-to-day basics. In the existing approach, there are various traditional machine learning classifiers for building a decision support system with limitations such as low detection rate and less feature selection. The important contents taken for building model from android applications like Intent Filter, Permission Signature, API Calls, and System commands are taken from the manifest file. The function parameters of various machine and deep learning classifiers like Nave Bayes, k-Nearest Neighbors (k-NN), Support Vector Machine (SVM), Ada Boost, and Multi-Layer Perceptron (MLP) are done for effective results. In our proposed work, we have used an unsupervised learning multilayer perceptron with multiple target labels and built a model with a better accuracy rate compared to logistic regression, and rank the best features for detection of applications and classify as malicious or benign can be used as threat model by online antivirus scanners.Keywords
Cite This Article
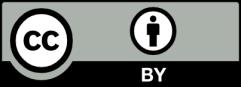