Open Access
ARTICLE
Deep Fake Detection Using Computer Vision-Based Deep Neural Network with Pairwise Learning
1 Department of Electronics and Communication Engineering, Anna University, University College of Engineering, Dindigul, 624622, India
2 Department of Computer Science and Engineering, Anna University, University College of Engineering, Dindigul, 624622, India
3 Department of Mathematics, Faculty of Science, Sana’a University, Sana’a 13509, Yemen
4 Department of Computer Science, College of Computers and Information Technology, Taif University, Taif, 21944, Saudi Arabia
5 Computer Sciences Department, College of Computer and Information Sciences, Princess Nourah bint Abdulrahman University (PNU), Riyadh, 11671, Saudi Arabia
6 Department of Mathematics, Faculty of Science, Mansoura University, Mansoura, 35516, Egypt
7 Department of Computational Mathematics, Science, and Engineering (CMSE), Michigan State University, East Lansing, MI, 48824, USA
* Corresponding Author: Mohamed Abouhawwash. Email:
Intelligent Automation & Soft Computing 2023, 35(2), 2449-2462. https://doi.org/10.32604/iasc.2023.030486
Received 27 March 2022; Accepted 27 April 2022; Issue published 19 July 2022
Abstract
Deep learning-based approaches are applied successfully in many fields such as deepFake identification, big data analysis, voice recognition, and image recognition. Deepfake is the combination of deep learning in fake creation, which states creating a fake image or video with the help of artificial intelligence for political abuse, spreading false information, and pornography. The artificial intelligence technique has a wide demand, increasing the problems related to privacy, security, and ethics. This paper has analyzed the features related to the computer vision of digital content to determine its integrity. This method has checked the computer vision features of the image frames using the fuzzy clustering feature extraction method. By the proposed deep belief network with loss handling, the manipulation of video/image is found by means of a pairwise learning approach. This proposed approach has improved the accuracy of the detection rate by 98% on various datasets.Keywords
Cite This Article
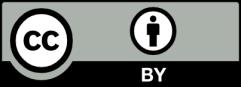