Open Access
ARTICLE
An Efficient Allocation for Lung Transplantation Using Ant Colony Optimization
Biomedical Systems and Informatics Engineering Department, Hijjawi for Engineering Technology, Yarmouk University, Irbid, 21163, Jordan
* Corresponding Author: Lina M. K. Al-Ebbini. Email:
Intelligent Automation & Soft Computing 2023, 35(2), 1971-1985. https://doi.org/10.32604/iasc.2023.030100
Received 18 March 2022; Accepted 20 April 2022; Issue published 19 July 2022
Abstract
A relationship between lung transplant success and many features of recipients’/donors has long been studied. However, modeling a robust model of a potential impact on organ transplant success has proved challenging. In this study, a hybrid feature selection model was developed based on ant colony optimization (ACO) and k-nearest neighbor (kNN) classifier to investigate the relationship between the most defining features of recipients/donors and lung transplant success using data from the United Network of Organ Sharing (UNOS). The proposed ACO-kNN approach explores the features space to identify the representative attributes and classify patients’ functional status (i.e., quality of life) after lung transplantation. The efficacy of the proposed model was verified using 3,684 records and 118 input features from the UNOS. The developed approach examined the reliability and validity of the lung allocation process. The results are promising regarding accuracy prediction to be 91.3% and low computational time, along with better decision capabilities, emphasizing the potential for automatic classification of the lung and other organs allocation processes. In addition, the proposed model recommends a new perspective on how medical experts and clinicians respond to uncertain and challenging lung allocation strategies. Having such ACO-kNN model, a medical professional can summarize information through the proposed method and make decisions for the upcoming transplants to allocate the donor organ.Keywords
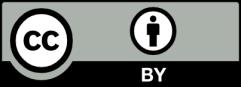