Open Access
ARTICLE
Fault Diagnosis in Robot Manipulators Using SVM and KNN
1 Laboratoire de Robotiques Parallélisme et Systèmes Embarquer, Département Automatique et Control, Université des Sciences et Technologie Houari Boumediene, Algiers, Algeria
2 Laboratoire de Recherche en Electrotechnique, Ecole Nationale Polytechnique, B.P 182, El-Harrach, 16200, Algiers, Algeria
3 Electrical Engineering Department, College of Engineering, Taif University, P. O. Box 11099, Taif, 21944, Saudi Arabia
* Corresponding Author: D. Maincer. Email:
Intelligent Automation & Soft Computing 2023, 35(2), 1957-1969. https://doi.org/10.32604/iasc.2023.029210
Received 27 February 2022; Accepted 14 April 2022; Issue published 19 July 2022
Abstract
In this paper, Support Vector Machine (SVM) and K-Nearest Neighbor (KNN) based methods are to be applied on fault diagnosis in a robot manipulator. A comparative study between the two classifiers in terms of successfully detecting and isolating the seven classes of sensor faults is considered in this work. For both classifiers, the torque, the position and the speed of the manipulator have been employed as the input vector. However, it is to mention that a large database is needed and used for the training and testing phases. The SVM method used in this paper is based on the Gaussian kernel with the parameters γ and the penalty margin parameter “C”, which were adjusted via the PSO algorithm to achieve a maximum accuracy diagnosis. Simulations were carried out on the model of a Selective Compliance Assembly Robot Arm (SCARA) robot manipulator, and the results showed that the Particle Swarm Optimization (PSO) increased the performance of the SVM algorithm with the 96.95% accuracy while the KNN algorithm achieved a correlation up to 94.62%. These results showed that the SVM algorithm with PSO was more precise than the KNN algorithm when was used in fault diagnosis on a robot manipulator.Keywords
Cite This Article
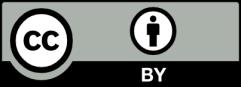
This work is licensed under a Creative Commons Attribution 4.0 International License , which permits unrestricted use, distribution, and reproduction in any medium, provided the original work is properly cited.