Open Access
ARTICLE
Enhanced Attention-Based Encoder-Decoder Framework for Text Recognition
School of Computing, SASTRA Deemed to be University, Thanjavur, 613401, India
* Corresponding Author: K. Joseph Abraham Sundar. Email:
Intelligent Automation & Soft Computing 2023, 35(2), 2071-2086. https://doi.org/10.32604/iasc.2023.029105
Received 25 February 2022; Accepted 19 April 2022; Issue published 19 July 2022
Abstract
Recognizing irregular text in natural images is a challenging task in computer vision. The existing approaches still face difficulties in recognizing irregular text because of its diverse shapes. In this paper, we propose a simple yet powerful irregular text recognition framework based on an encoder-decoder architecture. The proposed framework is divided into four main modules. Firstly, in the image transformation module, a Thin Plate Spline (TPS) transformation is employed to transform the irregular text image into a readable text image. Secondly, we propose a novel Spatial Attention Module (SAM) to compel the model to concentrate on text regions and obtain enriched feature maps. Thirdly, a deep bi-directional long short-term memory (Bi-LSTM) network is used to make a contextual feature map out of a visual feature map generated from a Convolutional Neural Network (CNN). Finally, we propose a Dual Step Attention Mechanism (DSAM) integrated with the Connectionist Temporal Classification (CTC) - Attention decoder to re-weights visual features and focus on the intra-sequence relationships to generate a more accurate character sequence. The effectiveness of our proposed framework is verified through extensive experiments on various benchmarks datasets, such as SVT, ICDAR, CUTE80, and IIIT5k. The performance of the proposed text recognition framework is analyzed with the accuracy metric. Demonstrate that our proposed method outperforms the existing approaches on both regular and irregular text. Additionally, the robustness of our approach is evaluated using the grocery datasets, such as GroZi-120, WebMarket, SKU-110K, and Freiburg Groceries datasets that contain complex text images. Still, our framework produces superior performance on grocery datasets.Keywords
Cite This Article
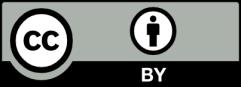