Open Access
ARTICLE
Multilevel Augmentation for Identifying Thin Vessels in Diabetic Retinopathy Using UNET Model
1 Faculty of Computer Science and Engineering, Sathyabama Institute of Science and Technology, Chennai, 600119, India
2 Department of Computer Science and Engineering, St. Joseph’s Institute of Technology, Chennai, 600119, India
* Corresponding Author: A. Deepak Kumar. Email:
Intelligent Automation & Soft Computing 2023, 35(2), 2273-2288. https://doi.org/10.32604/iasc.2023.028996
Received 22 February 2022; Accepted 12 April 2022; Issue published 19 July 2022
Abstract
Diabetic Retinopathy is a disease, which happens due to abnormal growth of blood vessels that causes spots on the vision and vision loss. Various techniques are applied to identify the disease in the early stage with different methods and parameters. Machine Learning (ML) techniques are used for analyzing the images and finding out the location of the disease. The restriction of the ML is a dataset size, which is used for model evaluation. This problem has been overcome by using an augmentation method by generating larger datasets with multidimensional features. Existing models are using only one augmentation technique, which produces limited features of dataset and also lacks in the association of those data during DR detection, so multilevel augmentation is proposed for analysis. The proposed method performs in two phases namely integrated augmentation model and dataset correlation (i.e. relationships). It eliminates over fitting problem by considering relevant dataset. This method is used for solving the Diabetic Retinopathy problem with a thin vessel identification using the UNET model. UNET based image segmentation achieves 98.3% accuracy when compared to RV-GAN and different UNET models with high detection rate.Keywords
Cite This Article
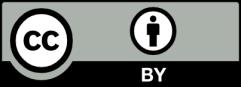
This work is licensed under a Creative Commons Attribution 4.0 International License , which permits unrestricted use, distribution, and reproduction in any medium, provided the original work is properly cited.