Open Access
ARTICLE
A Novel Outlier Detection with Feature Selection Enabled Streaming Data Classification
1 Anna University, Chennai, 600025, India
2 University College of Engineering, BIT Campus, Anna University, Tiruchirappalli, 620024, India
* Corresponding Author: R. Rajakumar. Email:
Intelligent Automation & Soft Computing 2023, 35(2), 2101-2116. https://doi.org/10.32604/iasc.2023.028889
Received 20 February 2022; Accepted 29 March 2022; Issue published 19 July 2022
Abstract
Due to the advancements in information technologies, massive quantity of data is being produced by social media, smartphones, and sensor devices. The investigation of data stream by the use of machine learning (ML) approaches to address regression, prediction, and classification problems have received considerable interest. At the same time, the detection of anomalies or outliers and feature selection (FS) processes becomes important. This study develops an outlier detection with feature selection technique for streaming data classification, named ODFST-SDC technique. Initially, streaming data is pre-processed in two ways namely categorical encoding and null value removal. In addition, Local Correlation Integral (LOCI) is used which is significant in the detection and removal of outliers. Besides, red deer algorithm (RDA) based FS approach is employed to derive an optimal subset of features. Finally, kernel extreme learning machine (KELM) classifier is used for streaming data classification. The design of LOCI based outlier detection and RDA based FS shows the novelty of the work. In order to assess the classification outcomes of the ODFST-SDC technique, a series of simulations were performed using three benchmark datasets. The experimental results reported the promising outcomes of the ODFST-SDC technique over the recent approaches.Keywords
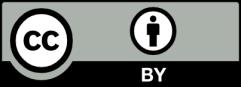