Open Access
ARTICLE
Evaluating Partitioning Based Clustering Methods for Extended Non-negative Matrix Factorization (NMF)
1 Guru Gobind Singh Indraprastha University, Delhi, India
2 Bhagwan Parshuram Institute of Technology, Delhi, India
* Corresponding Author: Neetika Bhandari. Email:
Intelligent Automation & Soft Computing 2023, 35(2), 2043-2055. https://doi.org/10.32604/iasc.2023.028368
Received 08 February 2022; Accepted 12 April 2022; Issue published 19 July 2022
Abstract
Data is humongous today because of the extensive use of World Wide Web, Social Media and Intelligent Systems. This data can be very important and useful if it is harnessed carefully and correctly. Useful information can be extracted from this massive data using the Data Mining process. The information extracted can be used to make vital decisions in various industries. Clustering is a very popular Data Mining method which divides the data points into different groups such that all similar data points form a part of the same group. Clustering methods are of various types. Many parameters and indexes exist for the evaluation and comparison of these methods. In this paper, we have compared partitioning based methods K-Means, Fuzzy C-Means (FCM), Partitioning Around Medoids (PAM) and Clustering Large Application (CLARA) on secure perturbed data. Comparison and identification has been done for the method which performs better for analyzing the data perturbed using Extended NMF on the basis of the values of various indexes like Dunn Index, Silhouette Index, Xie-Beni Index and Davies-Bouldin Index.Keywords
Cite This Article
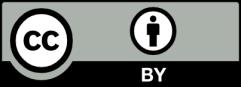
This work is licensed under a Creative Commons Attribution 4.0 International License , which permits unrestricted use, distribution, and reproduction in any medium, provided the original work is properly cited.