Open Access
ARTICLE
Ensemble Deep Learning with Chimp Optimization Based Medical Data Classification
1 Department of Computer Science and Information Systems, College of Applied Sciences, AlMaarefa University, Ad Diriyah, Riyadh, 13713, Kingdom of Saudi Arabia
2 Department of Computer Engineering, College of Computers and Information Technology, Taif University, Taif, 21944, Kingdom of Saudi Arabia
3 Department of Computing, Arabeast Colleges, Riyadh, 11583, Kingdom of Saudi Arabia
4 Department of Basic Medical Sciences, College of Medicine. Almaarefa University, AlMaarefa University, Ad Diriyah, Riyadh, 13713, Kingdom of Saudi Arabia
5 Department of Archives and Communication, King Faisal University, Al Ahsa, Hofuf, 31982, Kingdom of Saudi Arabia
* Corresponding Author: Ashit Kumar Dutta. Email:
Intelligent Automation & Soft Computing 2023, 35(2), 1643-1655. https://doi.org/10.32604/iasc.2023.027865
Received 27 January 2022; Accepted 07 March 2022; Issue published 19 July 2022
Abstract
Eye state classification acts as a vital part of the biomedical sector, for instance, smart home device control, drowsy driving recognition, and so on. The modifications in the cognitive levels can be reflected via transforming the electroencephalogram (EEG) signals. The deep learning (DL) models automated extract the features and often showcased improved outcomes over the conventional classification model in the recognition processes. This paper presents an Ensemble Deep Learning with Chimp Optimization Algorithm for EEG Eye State Classification (EDLCOA-ESC). The proposed EDLCOA-ESC technique involves min-max normalization approach as a pre-processing step. Besides, wavelet packet decomposition (WPD) technique is employed for the extraction of useful features from the EEG signals. In addition, an ensemble of deep sparse autoencoder (DSAE) and kernel ridge regression (KRR) models are employed for EEG Eye State classification. Finally, hyperparameters tuning of the DSAE model takes place using COA and thereby boost the classification results to a maximum extent. An extensive range of simulation analysis on the benchmark dataset is carried out and the results reported the promising performance of the EDLCOA-ESC technique over the recent approaches with maximum accuracy of 98.50%.Keywords
Cite This Article
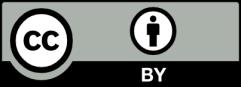