Open Access
ARTICLE
Using GAN Neural Networks for Super-Resolution Reconstruction of Temperature Fields
1 School of Artificial Intelligence, Nanjing University of Information Science & Technology, Nanjing, 210000, China
2 Unit 93117 of PLA, Nanjing, 210000, China
3 International Business Machines Corporation (IBM), New York, 100014, USA
4 School of Computer and Software, Nanjing University of Information Science & Technology, Nanjing, 210000, China
* Corresponding Author: Zhiwei Jiang. Email:
Intelligent Automation & Soft Computing 2023, 35(1), 941-956. https://doi.org/10.32604/iasc.2023.029644
Received 08 March 2022; Accepted 21 April 2022; Issue published 06 June 2022
Abstract
A Generative Adversarial Neural (GAN) network is designed based on deep learning for the Super-Resolution (SR) reconstruction task of temperature fields (comparable to downscaling in the meteorological field), which is limited by the small number of ground stations and the sparse distribution of observations, resulting in a lack of fineness of data. To improve the network’s generalization performance, the residual structure, and batch normalization are used. Applying the nearest interpolation method to avoid over-smoothing of the climate element values instead of the conventional Bicubic interpolation in the computer vision field. Sub-pixel convolution is used instead of transposed convolution or interpolation methods for up-sampling to speed up network inference. The experimental dataset is the European Centre for Medium-Range Weather Forecasts Reanalysis v5 (ERA5) with a bidirectional resolution of . On the other hand, the task aims to scale up the size by a factor of 8, which is rare compared to conventional methods. The comparison methods include traditional interpolation methods and a more widely used GAN-based network such as the SRGAN. The final experimental results show that the proposed scheme advances the performance of Root Mean Square Error (RMSE) by 37.25%, the Peak Signal-to-noise Ratio (PNSR) by 14.4%, and the Structural Similarity (SSIM) by 10.3% compared to the Bicubic Interpolation. For the traditional SRGAN network, a relatively obvious performance improvement is observed by experimental demonstration. Meanwhile, the GAN network can converge stably and reach the approximate Nash equilibrium for various initialization parameters to empirically illustrate the effectiveness of the method in the temperature fields.Keywords
Cite This Article
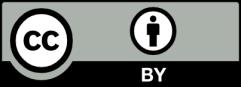
This work is licensed under a Creative Commons Attribution 4.0 International License , which permits unrestricted use, distribution, and reproduction in any medium, provided the original work is properly cited.