Open Access
ARTICLE
An Efficient ResNetSE Architecture for Smoking Activity Recognition from Smartwatch
1 Department of Computer Engineering, Image Information and Intelligence Laboratory, Faculty of Engineering, Mahidol University, Nakhon Pathom, 73170, Thailand
2 Department of Computer Engineering, School of Information and Communication Technology University of Phayao, Phayao, 56000, Thailand
3 Department of Mathematics, Faculty of Applied Science, King Mongkut’s University of Technology North Bangkok, Bangkok, 10800, Thailand
4 Intelligent and Nonlinear Dynamic Innovations Research Center, Science and Technology Research Institute, King Mongkut’s University of Technology North Bangkok, Bangkok 10800, Thailand
* Corresponding Author: Anuchit Jitpattanakul. Email:
Intelligent Automation & Soft Computing 2023, 35(1), 1245-1259. https://doi.org/10.32604/iasc.2023.028290
Received 07 February 2022; Accepted 20 March 2022; Issue published 06 June 2022
Abstract
Smoking is a major cause of cancer, heart disease and other afflictions that lead to early mortality. An effective smoking classification mechanism that provides insights into individual smoking habits would assist in implementing addiction treatment initiatives. Smoking activities often accompany other activities such as drinking or eating. Consequently, smoking activity recognition can be a challenging topic in human activity recognition (HAR). A deep learning framework for smoking activity recognition (SAR) employing smartwatch sensors was proposed together with a deep residual network combined with squeeze-and-excitation modules (ResNetSE) to increase the effectiveness of the SAR framework. The proposed model was tested against basic convolutional neural networks (CNNs) and recurrent neural networks (LSTM, BiLSTM, GRU and BiGRU) to recognize smoking and other similar activities such as drinking, eating and walking using the UT-Smoke dataset. Three different scenarios were investigated for their recognition performances using standard HAR metrics (accuracy, F1-score and the area under the ROC curve). Our proposed ResNetSE outperformed the other basic deep learning networks, with maximum accuracy of 98.63%.Keywords
Cite This Article
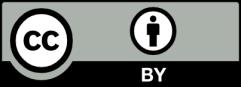
This work is licensed under a Creative Commons Attribution 4.0 International License , which permits unrestricted use, distribution, and reproduction in any medium, provided the original work is properly cited.