Open Access
ARTICLE
Deep Learning Based Autonomous Transport System for Secure Vehicle and Cargo Matching
1 Department of Electronics and Communication Engineering, Sona College of Technology, Salem, 636005, Tamil Nadu, India
2 Department of Data Science and Business Systems, School of Computing, SRM Institute of Science and Technology, Kattankulathur, 603203, Tamilnadu, India
3 Department of Electronics and Communication Engineering, K. Ramakrishnan College of Technology, Tiruchirapalli, 621112, Tamilnadu, India
4 Department of Electronics and Communication Engineering, K. Ramakrishnan College of Engineering, Tiruchirapalli, 621112, Tamilnadu, India
* Corresponding Author: T. Shanthi. Email:
Intelligent Automation & Soft Computing 2023, 35(1), 957-969. https://doi.org/10.32604/iasc.2023.027775
Received 25 January 2022; Accepted 11 March 2022; Issue published 06 June 2022
Abstract
The latest 6G improvements secured autonomous driving's realism in Intelligent Autonomous Transport Systems (IATS). Despite the IATS's benefits, security remains a significant challenge. Blockchain technology has grown in popularity as a means of implementing safe, dependable, and decentralised independent IATS systems, allowing for more utilisation of legacy IATS infrastructures and resources, which is especially advantageous for crowdsourcing technologies. Blockchain technology can be used to address security concerns in the IATS and to aid in logistics development. In light of the inadequacy of reliance and inattention to rights created by centralised and conventional logistics systems, this paper discusses the creation of a blockchain-based IATS powered by deep learning for secure cargo and vehicle matching (BDL-IATS). The BDL-IATS approach utilises Ethereum as the primary blockchain for storing private data such as order and shipment details. Additionally, the deep belief network (DBN) model is used to select suitable vehicles and goods for transportation. Additionally, the chaotic krill herd technique is used to tune the DBN model’s hyperparameters. The performance of the BDL-IATS technique is validated, and the findings are inspected under a variety of conditions. The simulation findings indicated that the BDL-IATS strategy outperformed recent state-of-the-art approaches.Keywords
Cite This Article
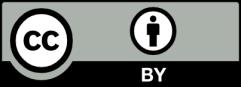
This work is licensed under a Creative Commons Attribution 4.0 International License , which permits unrestricted use, distribution, and reproduction in any medium, provided the original work is properly cited.