Open Access
ARTICLE
An Optimized Algorithm for Renewable Energy Forecasting Based on Machine Learning
1 College of Engineering at Wadi Addawaser, Prince Sattam bin Abdulaziz University, Wadi Aldawaser, 11991, Saudi Arabia
2 Electrical Engineering Department, Aswan Faculty of Engineering, Aswan University, Aswan, 81542, Egypt
3 Production Engineering and Mechanical Design Department, Faculty of Engineering, Mansoura University, Mansoura, 35516, Egypt
4 Department of Computer, College of Science and Arts in Ar Rass, Qassim University, Ar Rass, Saudi Arabia
5 Department of Electrical Engineering, University of Engineering and Technology, Peshawar, 814, Pakistan
* Corresponding Author: Ziad M. Ali. Email:
Intelligent Automation & Soft Computing 2023, 35(1), 755-767. https://doi.org/10.32604/iasc.2023.027568
Received 20 January 2022; Accepted 25 February 2022; Issue published 06 June 2022
Abstract
The large-scale application of renewable energy power generation technology brings new challenges to the operation of traditional power grids and energy management on the load side. Microgrid can effectively solve this problem by using its regulation and flexibility, and is considered to be an ideal platform. The traditional method of computing total transfer capability is difficult due to the central integration of wind farms. As a result, the differential evolution extreme learning machine is offered as a data mining approach for extracting operating rules for the total transfer capability of tie-lines in wind-integrated power systems. K-medoids clustering under the two-dimensional “wind power-load consumption” feature space is used to define representative operational scenarios initially. Then, using stochastic sampling and repetitive power flow, a knowledge base for total transfer capability operating rule mining is created. Then, a novel method is used to filter redundant characteristics and find features that are closely associated to the total transfer capability in order to decrease the ultra-high dimensionality of operational features. Finally, by feeding the training data into the proposed algorithm, the total transfer capability operation rules are derived from the knowledge base. It can be seen that, the proposed algorithm can optimize the system performance with good accuracy and generality, according to numerical data.Keywords
Cite This Article
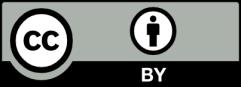
This work is licensed under a Creative Commons Attribution 4.0 International License , which permits unrestricted use, distribution, and reproduction in any medium, provided the original work is properly cited.