Open Access
ARTICLE
Optimized ANFIS Model for Stable Clustering in Cognitive Radio Network
1 Department of Information Technology, Velammal Institutute of Technology, Chennai, 600066, Tamilnadu, India
2 Department of Electronics and Communication Engineering, Velammal Engineering College, Chennai, 600040, Tamilnadu, India
* Corresponding Author: C. Ambhika. Email:
Intelligent Automation & Soft Computing 2023, 35(1), 827-838. https://doi.org/10.32604/iasc.2023.026832
Received 05 January 2022; Accepted 13 March 2022; Issue published 06 June 2022
Abstract
With the demand for wireless technology, Cognitive Radio (CR) technology is identified as a promising solution for effective spectrum utilization. Connectivity and robustness are the two main difficulties in cognitive radio networks due to their dynamic nature. These problems are solved by using clustering techniques which group the cognitive users into logical groups. The performance of clustering in cognitive network purely depends on cluster head selection and parameters considered for clustering. In this work, an adaptive neuro-fuzzy inference system (ANFIS) based clustering is proposed for the cognitive network. The performance of ANFIS improved using hybrid particle swarm and whale optimization algorithms for parameter tuning called PSWO. The consequent and antecedent parameters of ANFIS model are tuned by PSWO. The proper cluster heads from the network are identified using optimized ANFIS. The proposed optimized ANFIS based clustering model is analyzed in terms of number of clusters, number of common channels, reclustering rate and stability period. Simulation results indicate that proposed clustering effectively increase the stability of cluster with reduced communication overhead compared to other conventional clustering algorithms.Keywords
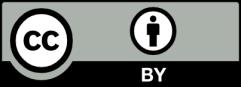