Open Access
ARTICLE
Hybrid Deep Learning Based Attack Detection for Imbalanced Data Classification
1 Department of Computer Science, Faculty of Computing and Information Technology, King Abdulaziz University, Jeddah, Saudi Arabia
2 Department of Computer Science, Faculty of Computer Science and Engineering, University of Hail, Hail, Saudi Arabia
* Corresponding Author: Rasha Almarshdi. Email:
Intelligent Automation & Soft Computing 2023, 35(1), 297-320. https://doi.org/10.32604/iasc.2023.026799
Received 04 January 2022; Accepted 23 February 2022; Issue published 06 June 2022
Abstract
Internet of Things (IoT) is the most widespread and fastest growing technology today. Due to the increasing of IoT devices connected to the Internet, the IoT is the most technology under security attacks. The IoT devices are not designed with security because they are resource constrained devices. Therefore, having an accurate IoT security system to detect security attacks is challenging. Intrusion Detection Systems (IDSs) using machine learning and deep learning techniques can detect security attacks accurately. This paper develops an IDS architecture based on Convolutional Neural Network (CNN) and Long Short-Term Memory (LSTM) deep learning algorithms. We implement our model on the UNSW-NB15 dataset which is a new network intrusion dataset that categorizes the network traffic into normal and attacks traffic. In this work, interpolation data preprocessing is used to compute the missing values. Also, the imbalanced data problem is solved using a synthetic data generation method. Extensive experiments have been implemented to compare the performance results of the proposed model (CNN+LSTM) with a basic model (CNN only) using both balanced and imbalanced dataset. Also, with some state-of-the-art machine learning classifiers (Decision Tree (DT) and Random Forest (RF)) using both balanced and imbalanced dataset. The results proved the impact of the balancing technique. The proposed hybrid model with the balance technique can classify the traffic into normal class and attack class with reasonable accuracy (92.10%) compared with the basic CNN model (89.90%) and the machine learning (DT 88.57% and RF 90.85%) models. Moreover, comparing the proposed model results with the most related works shows that the proposed model gives good results compared with the related works that used the balance techniques.Keywords
Cite This Article
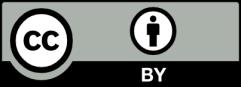
This work is licensed under a Creative Commons Attribution 4.0 International License , which permits unrestricted use, distribution, and reproduction in any medium, provided the original work is properly cited.