Open Access
ARTICLE
An Intrusion Detection System for SDN Using Machine Learning
Department of Computer Science and Engineering, College of Engineering, Guindy, Anna University, Chennai, Tamilnadu, India
* Corresponding Author: G. Logeswari. Email:
Intelligent Automation & Soft Computing 2023, 35(1), 867-880. https://doi.org/10.32604/iasc.2023.026769
Received 04 January 2022; Accepted 16 February 2022; Issue published 06 June 2022
Abstract
Software Defined Networking (SDN) has emerged as a promising and exciting option for the future growth of the internet. SDN has increased the flexibility and transparency of the managed, centralized, and controlled network. On the other hand, these advantages create a more vulnerable environment with substantial risks, culminating in network difficulties, system paralysis, online banking frauds, and robberies. These issues have a significant detrimental impact on organizations, enterprises, and even economies. Accuracy, high performance, and real-time systems are necessary to achieve this goal. Using a SDN to extend intelligent machine learning methodologies in an Intrusion Detection System (IDS) has stimulated the interest of numerous research investigators over the last decade. In this paper, a novel HFS-LGBM IDS is proposed for SDN. First, the Hybrid Feature Selection algorithm consisting of two phases is applied to reduce the data dimension and to obtain an optimal feature subset. In the first phase, the Correlation based Feature Selection (CFS) algorithm is used to obtain the feature subset. The optimal feature set is obtained by applying the Random Forest Recursive Feature Elimination (RF-RFE) in the second phase. A LightGBM algorithm is then used to detect and classify different types of attacks. The experimental results based on NSL-KDD dataset show that the proposed system produces outstanding results compared to the existing methods in terms of accuracy, precision, recall and f-measure.Keywords
Cite This Article
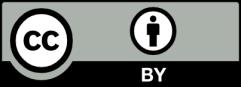
This work is licensed under a Creative Commons Attribution 4.0 International License , which permits unrestricted use, distribution, and reproduction in any medium, provided the original work is properly cited.