Open Access
ARTICLE
Impact of Data Quality on Question Answering System Performances
LASTIMI Laboratory, Mohammadia School of Engineers, Mohammed V University in Rabat, Morocco
* Corresponding Author: Rachid Karra. Email:
Intelligent Automation & Soft Computing 2023, 35(1), 335-349. https://doi.org/10.32604/iasc.2023.026695
Received 02 January 2022; Accepted 15 February 2022; Issue published 06 June 2022
Abstract
In contrast with the research of new models, little attention has been paid to the impact of low or high-quality data feeding a dialogue system. The present paper makes the first attempt to fill this gap by extending our previous work on question-answering (QA) systems by investigating the effect of misspelling on QA agents and how context changes can enhance the responses. Instead of using large language models trained on huge datasets, we propose a method that enhances the model's score by modifying only the quality and structure of the data feed to the model. It is important to identify the features that modify the agent performance because a high rate of wrong answers can make the students lose their interest in using the QA agent as an additional tool for distant learning. The results demonstrate the accuracy of the proposed context simplification exceeds 85%. These findings shed light on the importance of question data quality and context complexity construct as key dimensions of the QA system. In conclusion, the experimental results on questions and contexts showed that controlling and improving the various aspects of data quality around the QA system can significantly enhance his robustness and performance.Keywords
Cite This Article
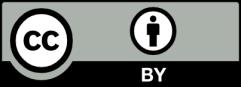
This work is licensed under a Creative Commons Attribution 4.0 International License , which permits unrestricted use, distribution, and reproduction in any medium, provided the original work is properly cited.