Open Access
ARTICLE
Metaheuristics with Optimal Deep Transfer Learning Based Copy-Move Forgery Detection Technique
1 Department of Information Technology, Hindusthan College of Engineering and Technology, Coimbatore, 641032, Tamilnadu, India
2 Department of Electrical and Electronics Engineering, Hindusthan College of Engineering and Technology, Coimbatore, 641032, Tamilnadu, India
* Corresponding Author: C. D. Prem Kumar. Email:
Intelligent Automation & Soft Computing 2023, 35(1), 881-899. https://doi.org/10.32604/iasc.2023.025766
Received 03 December 2021; Accepted 23 January 2022; Issue published 06 June 2022
Abstract
The extensive availability of advanced digital image technologies and image editing tools has simplified the way of manipulating the image content. An effective technique for tampering the identification is the copy-move forgery. Conventional image processing techniques generally search for the patterns linked to the fake content and restrict the usage in massive data classification. Contrastingly, deep learning (DL) models have demonstrated significant performance over the other statistical techniques. With this motivation, this paper presents an Optimal Deep Transfer Learning based Copy Move Forgery Detection (ODTL-CMFD) technique. The presented ODTL-CMFD technique aims to derive a DL model for the classification of target images into the original and the forged/tampered, and then localize the copy moved regions. To perform the feature extraction process, the political optimizer (PO) with Mobile Networks (MobileNet) model has been derived for generating a set of useful vectors. Finally, an enhanced bird swarm algorithm (EBSA) with least square support vector machine (LS-SVM) model has been employed for classifying the digital images into the original or the forged ones. The utilization of the EBSA algorithm helps to properly modify the parameters contained in the Multiclass Support Vector Machine (MSVM) technique and thereby enhance the classification performance. For ensuring the enhanced performance of the ODTL-CMFD technique, a series of simulations have been performed against the benchmark MICC-F220, MICC-F2000, and MICC-F600 datasets. The experimental results have demonstrated the improvised performance of the ODTL-CMFD approach over the other techniques in terms of several evaluation measures.Keywords
Cite This Article
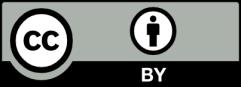
This work is licensed under a Creative Commons Attribution 4.0 International License , which permits unrestricted use, distribution, and reproduction in any medium, provided the original work is properly cited.