Open Access
ARTICLE
Hybrid Convolutional Neural Network and Long Short-Term Memory Approach for Facial Expression Recognition
1 Department of Computer Science & Engineering, Kongu Engineering College, Perundurai, Erode, 638060, Tamil Nadu, India
2 Department of Computer Science & Engineering, K.S.R. College of Engineering, Tiruchengode, 637215, Tamil Nadu, India
* Corresponding Author: M. N. Kavitha. Email:
Intelligent Automation & Soft Computing 2023, 35(1), 689-704. https://doi.org/10.32604/iasc.2023.025437
Received 23 November 2021; Accepted 27 February 2022; Issue published 06 June 2022
Abstract
Facial Expression Recognition (FER) has been an important field of research for several decades. Extraction of emotional characteristics is crucial to FERs, but is complex to process as they have significant intra-class variances. Facial characteristics have not been completely explored in static pictures. Previous studies used Convolution Neural Networks (CNNs) based on transfer learning and hyperparameter optimizations for static facial emotional recognitions. Particle Swarm Optimizations (PSOs) have also been used for tuning hyperparameters. However, these methods achieve about 92 percent in terms of accuracy. The existing algorithms have issues with FER accuracy and precision. Hence, the overall FER performance is degraded significantly. To address this issue, this work proposes a combination of CNNs and Long Short-Term Memories (LSTMs) called the HCNN-LSTMs (Hybrid CNNs and LSTMs) approach for FERs. The work is evaluated on the benchmark dataset, Facial Expression Recog Image Ver (FERC). Viola-Jones (VJ) algorithms recognize faces from preprocessed images followed by HCNN-LSTMs feature extractions and FER classifications. Further, the success rate of Deep Learning Techniques (DLTs) has increased with hyperparameter tunings like epochs, batch sizes, initial learning rates, regularization parameters, shuffling types, and momentum. This proposed work uses Improved Weight based Whale Optimization Algorithms (IWWOAs) to select near-optimal settings for these parameters using best fitness values. The experimental findings demonstrated that the proposed HCNN-LSTMs system outperforms the existing methods.Keywords
Cite This Article
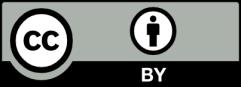
This work is licensed under a Creative Commons Attribution 4.0 International License , which permits unrestricted use, distribution, and reproduction in any medium, provided the original work is properly cited.