Open Access
ARTICLE
Attention Weight is Indispensable in Joint Entity and Relation Extraction
1 Key Laboratory of Intelligen Computing and Information Processing, Ministry of Education, Computer science College of Xiangtan University, Xiangtan, 411100, China
2 Department of Computer Science, University of Georgia, Athens, USA
* Corresponding Author: Jianquan Ouyang. Email:
Intelligent Automation & Soft Computing 2022, 34(3), 1707-1723. https://doi.org/10.32604/iasc.2022.028352
Received 08 February 2022; Accepted 11 April 2022; Issue published 25 May 2022
Abstract
Joint entity and relation extraction (JERE) is an important foundation for unstructured knowledge extraction in natural language processing (NLP). Thus, designing efficient algorithms for it has become a vital task. Although existing methods can efficiently extract entities and relations, their performance should be improved. In this paper, we propose a novel model called Attention and Span-based Entity and Relation Transformer (ASpERT) for JERE. First, differing from the traditional approach that only considers the last hidden layer as the feature embedding, ASpERT concatenates the attention head information of each layer with the information of the last hidden layer by using an attentional contribution degree algorithm, so as to remain the key information of the original sentence in a deep transferring of the pre-trained model. Second, considering the unstable performance of the linear span classification and width embedding structure of the SpERT, ASpERT uses a multilayer perceptron (MLP) and softmax-based span classification structure. Ablation experiments on the feature embedding and span classification structures both show better performances than SpERT’s. Moreover, the proposed model achieved desired results on three widely-used domain datasets (SciERC, CoNLL04, and ADE) and outperforms the current state-of-the-art model on SciERC. Specifically, the F1 score on SciERC is 52.30%, that on CoNLL04 is 71.66%, and that on ADE is 82.76%.Keywords
Cite This Article
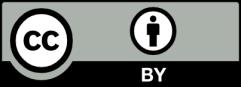