Open Access
ARTICLE
Sport-Related Activity Recognition from Wearable Sensors Using Bidirectional GRU Network
1 Department of Computer Engineering, School of Information and Communication Technology, University of Phayao, Phayao, 56000, Thailand
2 Intelligent and Nonlinear Dynamic Innovations Research Center, Department of Mathematics, Faculty of Applied Science, King Mongkut’s University of Technology North Bangkok, Bangkok, 10800, Thailand
* Corresponding Author: Anuchit Jitpattanakul. Email:
Intelligent Automation & Soft Computing 2022, 34(3), 1907-1925. https://doi.org/10.32604/iasc.2022.027233
Received 13 January 2022; Accepted 15 February 2022; Issue published 25 May 2022
Abstract
Numerous learning-based techniques for effective human activity recognition (HAR) have recently been developed. Wearable inertial sensors are critical for HAR studies to characterize sport-related activities. Smart wearables are now ubiquitous and can benefit people of all ages. HAR investigations typically involve sensor-based evaluation. Sport-related activities are unpredictable and have historically been classified as complex, with conventional machine learning (ML) algorithms applied to resolve HAR issues. The efficiency of machine learning techniques in categorizing data is limited by the human-crafted feature extraction procedure. A deep learning model named MBiGRU (multimodal bidirectional gated recurrent unit) neural network was proposed to recognize everyday sport-related actions, with the publicly accessible UCI-DSADS dataset utilized as a benchmark to compare the effectiveness of the proposed deep learning network against other deep learning architectures (CNNs and GRUs). Experiments were performed to quantify four evaluation criteria as accuracy, precision, recall and F1-score. Following a 10-fold cross-validation approach, the experimental findings indicated that the MBiGRU model presented superior accuracy of 99.55% against other benchmark deep learning networks. The available evidence was also evaluated to explore ways to enhance the proposed model and training procedure.Keywords
Cite This Article
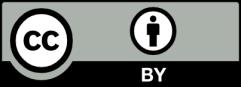
This work is licensed under a Creative Commons Attribution 4.0 International License , which permits unrestricted use, distribution, and reproduction in any medium, provided the original work is properly cited.