Open Access
ARTICLE
Deep Learning Based Distributed Intrusion Detection in Secure Cyber Physical Systems
1 Department of Electronics and Communication Engineering, University College of Engineering, BIT Campus, Anna University, Tiruchirapalli, 620025, India
2 Department of Electronics and Instrumentation Engineering, Kongu Engineering College, Erode, 638060, India
3 Department of Electronics and Communication Engineering, Gokaraju Rangaraju Institute of Engineering and Technology, Hyderabad, 500090, India
4 Department of Biomedical Engineering, Faculty of Engineering, Karpagam Academy of Higher Education, Coimbatore, 641021, India
* Corresponding Author: P. Ramadevi. Email:
Intelligent Automation & Soft Computing 2022, 34(3), 2067-2081. https://doi.org/10.32604/iasc.2022.026377
Received 23 December 2021; Accepted 16 February 2022; Issue published 25 May 2022
Abstract
Cyber Physical Systems (CPSs) are network systems containing cyber (computation, communication) and physical (sensors, actuators) components that interact with each other through feedback loop with the help of human intervention. The dynamic and disseminated characteristics of CPS environment makes it vulnerable to threats that exist in virtualization process. Due to this, several security issues are presented in CPS. In order to address the challenges, there is a need exists to extend the conventional security solutions such as Intrusion Detection Systems (IDS) to handle high speed network data traffic and adaptive network pattern in cloud. Additionally, the identification of feasible network traffic characteristics is the main issue in precise detection of attacks in the network. With this motivation, the current research paper presents an Optimal Deep Belief Network-based distributed Intrusion Detection System (ODBN-IDS) for secure CPS environment. The proposed model pre-process the cloud network traffic data to improve its quality to next level. Here, a Binary Flower Pollination Algorithm (BFPA) is employed for feature selection process. The attained characteristics are used in optimal Deep Belief Networks (DBN) to detect the presence of intrusion in cloud data and produce alarms, in case of presence of intrusions. Equilibrium Optimizer Algorithm (EOA) is used to fine tune the hyperparameters in DBN model. A detailed set of simulations was conducted on benchmark datasets and the analysis results were compared. A detailed comparison was conducted for various models to satisfy the security requirements of cloud network and the results established the supremacy of the proposed ODBN-IDS model.Keywords
Cite This Article
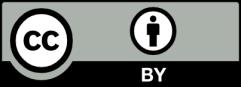
This work is licensed under a Creative Commons Attribution 4.0 International License , which permits unrestricted use, distribution, and reproduction in any medium, provided the original work is properly cited.