Open Access
ARTICLE
Multiple Events Detection Using Context-Intelligence Features
1 Department of Computer Science and Software Engineering, Al Ain University, Al Ain, 15551, UAE
2 Department of Computer Science, Air University, Islamabad, Pakistan
3 Department of Computer Science, College of Computer, Qassim University, Buraydah, 51452, Saudi Arabia
4 Department of Humanities and Social Science, Al Ain University, Al Ain, 15551, UAE
5 Department of Human-Computer Interaction, Hanyang University, Ansan, 15588, Korea
* Corresponding Author: Kibum Kim. Email:
Intelligent Automation & Soft Computing 2022, 34(3), 1455-1471. https://doi.org/10.32604/iasc.2022.025013
Received 08 November 2021; Accepted 13 January 2022; Issue published 25 May 2022
Abstract
Event detection systems are mainly used to observe and monitor human behavior via red green blue (RGB) images and videos. Event detection using RGB images is one of the challenging tasks of the current era. Human detection, position and orientation of human body parts in RGB images is a critical phase for numerous systems models. In this research article, the detection of human body parts by extracting context-aware energy features for event recognition is described. For this, silhouette extraction, estimation of human body parts, and context-aware features are extracted. To optimize the context-intelligence vector, we applied an artificial intelligence-based self-organized map (SOM) while a genetic algorithm (GA) is applied for multiple event detection. The experimental results on challenging RGB images and video-based datasets were promising. Three datasets were used. Event recognition and body parts detection accuracy rates for the University of central Florida’s (UCF) dataset were 88.88% and 86.75% respectively. 90.0% and 87.37% for event recognition and body parts detection were achieved over the University of Texas (UT) dataset. 87.89% and 85.87% for event recognition and body parts detection were achieved for the sports videos in the wild (SVW) dataset. The proposed system performs better than other current state-of-the-art approaches in terms of body parts and event detection and recognition outcomes.Keywords
Cite This Article
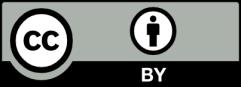
This work is licensed under a Creative Commons Attribution 4.0 International License , which permits unrestricted use, distribution, and reproduction in any medium, provided the original work is properly cited.