Open Access
ARTICLE
Shrinkage Linear with Quadratic Gaussian Discriminant Analysis for Big Data Classification
1 Department of Computer Science and Engineering, Kongu Engineering College, Erode, 638060, Tamilnadu, India
2 Department of Applied Cybernetics, Faculty of Science, University of Hradec Králové, Hradec Králové, 50003, Czech Republic
3 Department of Information Technology, College of Computers and Information Technology, Taif University, Taif, 21944, Saudi Arabia
4 Department of Mathematics, Faculty of Science, Mansoura University, Mansoura, 35516, Egypt
5 Department of Computational Mathematics, Science, and Engineering (CMSE), Michigan State University, East Lansing, 48824, MI, USA
* Corresponding Author: Mohamed Abouhawwash. Email:
Intelligent Automation & Soft Computing 2022, 34(3), 1803-1818. https://doi.org/10.32604/iasc.2022.024539
Received 21 October 2021; Accepted 05 February 2022; Issue published 25 May 2022
Abstract
Generation of massive data is increasing in big data industries due to the evolution of modern technologies. The big data industries include data source from sensors, Internet of Things, digital and social media. In particular, these big data systems consist of data extraction, preprocessing, integration, analysis, and visualization mechanism. The data encountered from the sources are redundant, incomplete and conflict. Moreover, in real time applications, it is a tedious process for the interpretation of all the data from different sources. In this paper, the gathered data are preprocessed to handle the issues such as redundant, incomplete and conflict. For that, it is proposed to have a generalized dimensionality reduction technique called Shrinkage Linear Discriminate Analysis (SLDA). As a result, the Shrinkage Linear Discriminate Analysis (LDA) will improve the performance of the classifier with generalization. Even though, dimensionality reduction systems improve the performance of the classifier, the irrelevant features get degraded by the performance of the system further. Hence, the relevant and the most important features are selected using Pearson correlation-based feature selection technique which selects the subset of correlated features for improving the performance of the classification system. The selected features are classified using the proposed Quadratic-Gaussian Discriminant Analysis (QGDA) classifier. The proposed evolution techniques are tested with the localization and the cover data sets from machine learning University of California Irvine (UCI) repository. In addition to that, the proposed techniques on datasets are evaluated with the evaluation metrics and compared to the other similar methods which prove the efficiency of the proposed classification system. It has achieved better performance. The acquired accuracy is over 91% for all the experiment on these datasets. Based on the results evaluated in terms of training percentage and mapper, it is meaningful to conclude that the proposed method could be used for big data classification.Keywords
Cite This Article
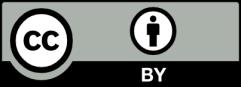