Open Access
ARTICLE
Dynamic Selection of Optional Feature for Object Detection
1 Science and Technology Industry Department, Nanjing University of Information Science & Technology, Nanjing, 210044, China
2 School of Computer & Software, Nanjing University of Information Science & Technology, Nanjing, 210044, China
3 Science and Technology Industry Department, Nanjing University of Information Science & Technology, Nanjing, 210044, China
4 Harbor Branch Oceanographic Institute, Florida Atlantic University, USA
* Corresponding Author: Tingjuan Zhang. Email:
Intelligent Automation & Soft Computing 2022, 34(2), 927-940. https://doi.org/10.32604/iasc.2022.026847
Received 05 January 2022; Accepted 16 February 2022; Issue published 03 May 2022
Abstract
To obtain the most intuitive pedestrian target detection results and avoid the impact of motion pose uncertainty on real-time detection, a pedestrian target detection system based on a convolutional neural network was designed. Dynamic Selection of Optional Feature (DSOF) module and a center branch were proposed in this paper, and the target was detected by an anchor-free method. Although almost all the most advanced target detectors use pre-defined anchor boxes to run through the possible positions, scales, and aspect ratios of search targets, their effectualness, and generalization ability are also limited by the anchor boxes. Most anchor-based detectors use heuristically guided anchor frames. Such a design is difficult to detect objects of different types and sizes, especially objects with highly overlapping boundaries. To solve this problem, the DSOF module is proposed in this paper, which selects for each instance the most appropriate feature layer through automatic feature selection. After using multi-level prediction, stacks of low-grade prediction bounding boxes will be generated far away from the target center. To eliminate these low-grade detections, we introduce a new center branch to predict the deviation of a pixel from its corresponding bounding box. This score is used to reduce the weight of the low-grade detection bounding box and merge the detection sequences into the Non-Maximum Suppression (NMS).Keywords
Cite This Article
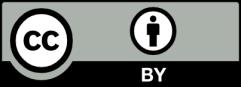
This work is licensed under a Creative Commons Attribution 4.0 International License , which permits unrestricted use, distribution, and reproduction in any medium, provided the original work is properly cited.