Open Access
ARTICLE
Adaptive Resource Allocation Neural Network-Based Mammogram Image Segmentation and Classification
Department of Electronics and Communication Engineering, Government College of Engineering, Salem, 636011, India
* Corresponding Author: G. Kavithaa. Email:
Intelligent Automation & Soft Computing 2022, 34(2), 877-893. https://doi.org/10.32604/iasc.2022.025982
Received 11 December 2021; Accepted 18 January 2022; Issue published 03 May 2022
Abstract
Image processing innovations assume a significant part in diagnosing and distinguishing diseases and monitoring these diseases’ quality. In Medical Images, detection of breast cancer in its earlier stage is most important in this field. Because of the low contrast and uncertain design of the tumor cells in breast images, it is still challenging to classify breast tumors only by visual testing by the radiologists. Hence, improvement of computer-supported strategies has been introduced for breast cancer identification. This work presents an efficient computer-assisted method for breast cancer classification of digital mammograms using Adaptive Resource Allocation Network (ARAN). At first, breast cancer images were taken as input, preprocessing step is utilized to eliminate the noise and unimportant data from the image utilizing a Butterworth filter. Adaptive histogram equalization is utilized to improve the contrast of the image. Multimodal clustering segmentation has been applied, and Tetrolet transformation based feature extraction is applied at various levels, based on this, data classification is implemented. For exact classification, ARAN is utilized to predict if the patient is influenced by breast cancer. Compared with other current research techniques, the proposed strategy predicts the results efficiently. The overall accuracy of ARAN-based mammogram classification is 93.3%.Keywords
Cite This Article
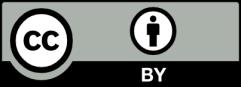
This work is licensed under a Creative Commons Attribution 4.0 International License , which permits unrestricted use, distribution, and reproduction in any medium, provided the original work is properly cited.