Open Access
ARTICLE
Automatic Annotation Performance of TextBlob and VADER on Covid Vaccination Dataset
Information Systems Department, College of Computer and Information Sciences, Imam Mohammad Ibn Saud Islamic University (IMSIU), Riyadh, 11432, Saudi Arabia
* Corresponding Author: Abdul Khader Jilani Saudagar. Email:
Intelligent Automation & Soft Computing 2022, 34(2), 1311-1331. https://doi.org/10.32604/iasc.2022.025861
Received 07 December 2021; Accepted 20 January 2022; Issue published 03 May 2022
Abstract
With the recent boom in the corpus size of sentiment analysis tasks, automatic annotation is poised to be a necessary alternative to manual annotation for generating ground truth dataset labels. This article aims to investigate and validate the performance of two widely used lexicon-based automatic annotation approaches, TextBlob and Valence Aware Dictionary and Sentiment Reasoner (VADER), by comparing them with manual annotation. The dataset of 5402 Arabic tweets was annotated manually, containing 3124 positive tweets, 1463 negative tweets, and 815 neutral tweets. The tweets were translated into English so that TextBlob and VADER could be used for their annotation. TextBlob and VADER automatically classified the tweets to positive, negative, and neutral sentiments and compared them with manual annotation. This study shows that automatic annotation cannot be trusted as the gold standard for annotation. In addition, the study discussed many drawbacks and limitations of automatic annotation using lexicon-based algorithms. The highest level of accuracies of 75% and 70% were achieved by TextBlob and VADER, respectively.Keywords
Cite This Article
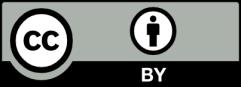